by Joche Ojeda | Mar 6, 2025 | Visual Studio
Visual Studio Sign-In Issues: A Simple Fix
For a long time, I had a problem with my Visual Studio installation. I was unable to sign in with different accounts or change my account. I tried resetting my computer to the default settings, but the issue persisted.
In December, I bought a new Microsoft Surface, which basically fixed the problem. However, I still have my own computer – a really good MSI gaming machine with 64 gigabytes of RAM. I decided to use it as a secondary computer, but the main problem remained: even after resetting my Windows setup to default and cleaning all programs, I was unable to sign in with a different account. I was essentially stuck.
Today, I decided to tackle this issue. It’s been a really busy week (it’s Thursday already), but I’ve accomplished a lot, including finishing two Udemy courses on Semantic Kernel and Microsoft AI Extensions. Having some free time, I played around with my old computer to see if I could fix it – and fortunately, I was able to!
The Solution
Here’s what I did to fix the sign-in problem:
- Go to Visual Studio Options > Environment > Accounts
- Change “Add and reauthenticate accounts using:” from “Windows authentication broker” to something else like “Embedded web browser” or “System browser”
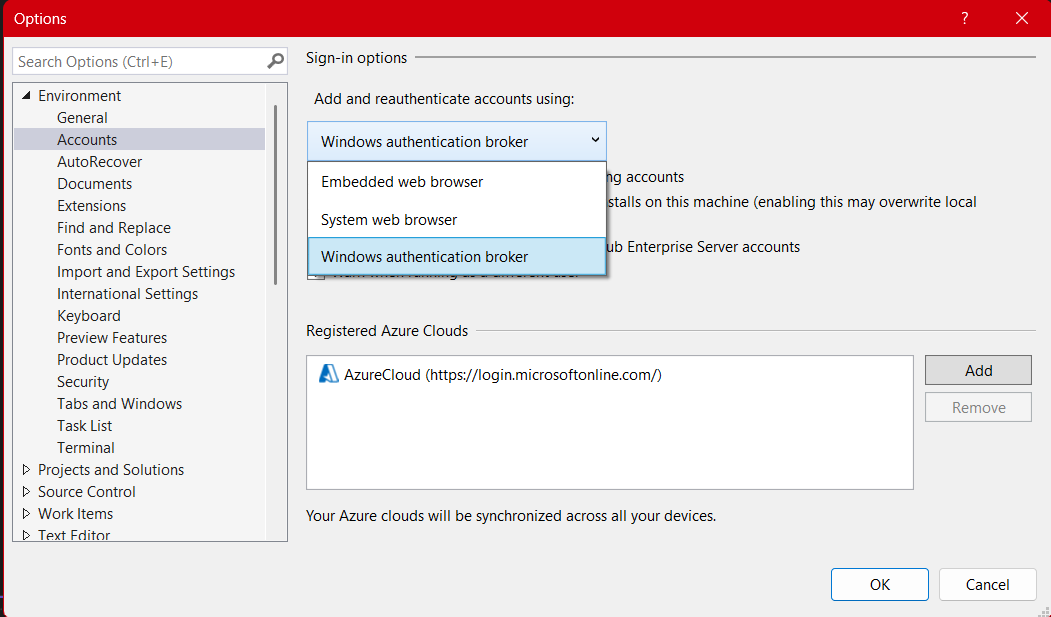
After changing to System browser or Embedded web browser, I was able to complete the entire sign-in flow without issues.
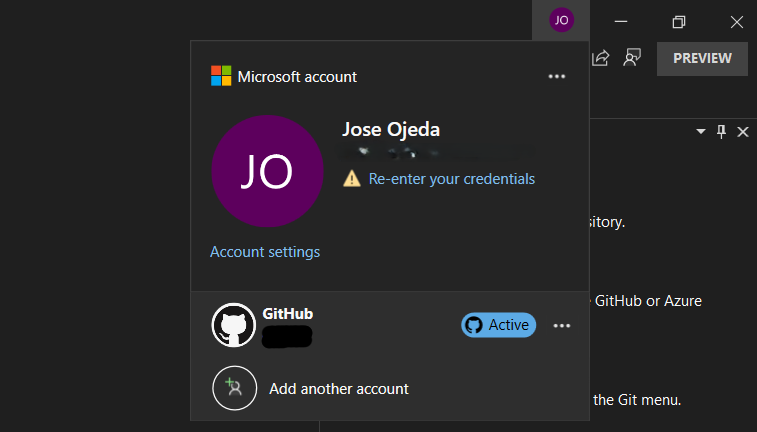
If you’re experiencing similar problems with Visual Studio account authentication, give this simple fix a try!
by Joche Ojeda | Mar 5, 2025 | C#, dotnet, Uno Platform
Exploring the Uno Platform: Handling Unsafe Code in Multi-Target Applications
This last weekend I wanted to do a technical experiment as I always do when I have some free time. I decided there was something new I needed to try and see if I could write about. The weekend turned out to be a beautiful surprise as I went back to test the Uno platform – a multi-OS, multi-target UI framework that generates mobile applications, desktop applications, web applications, and even Linux applications.
The idea of Uno is a beautiful concept, but for a long time, the tooling wasn’t quite there. I had made it work several times in the past, but after an update or something in Visual Studio, the setup would break and applications would become basically impossible to compile. That seems to no longer be the case!
Last weekend, I set up Uno on two different computers: my new Surface laptop with an ARM type of processor (which can sometimes be tricky for some tools) and my old MSI with an x64 type of processor. I was thrilled that the setup was effortless on both machines.
After the successful setup, I decided to download the entire Uno demo repository and start trying out the demos. However, for some reason, they didn’t compile. I eventually realized there was a problem with generated code during compilation time that turned out to be unsafe code. Here are my findings about how to handle the unsafe code that is generated.
AllowUnsafeBlocks Setting in Project File
I discovered that this setting was commented out in the Navigation.csproj file:
<!--<AllowUnsafeBlocks>true</AllowUnsafeBlocks>-->
When uncommented, this setting allows the use of unsafe code blocks in your .NET 8 Uno Platform project. To enable unsafe code, you need to remove the comment markers from this line in your project file.
Why It’s Needed
The <AllowUnsafeBlocks>true</AllowUnsafeBlocks> setting is required whenever you want to use “unsafe” code in C#. By default, C# is designed to be memory-safe, preventing direct memory manipulation that could lead to memory corruption, buffer overflows, or security vulnerabilities. When you add this setting to your project file, you’re explicitly telling the compiler to allow portions of code marked with the unsafe keyword.
Unsafe code lets you work with pointers and perform direct memory operations, which can be useful for:
- Performance-critical operations
- Interoperability with native code
- Direct memory manipulation
What Makes Code “Unsafe”
Code is considered “unsafe” when it bypasses .NET’s memory safety guarantees. Specifically, unsafe code includes:
- Pointer operations: Using the * and -> operators with memory addresses
- Fixed statements: Pinning managed objects in memory so their addresses don’t change during garbage collection
- Sizeof operator: Getting the size of a type in bytes
- Stackalloc keyword: Allocating memory on the stack instead of the heap
Example of Unsafe Code
Here’s an example of unsafe code that might be generated:
unsafe
{
int[] numbers = new int[] { 10, 20, 30, 40, 50 };
// UNSAFE: Pinning an array in memory and getting direct pointer
fixed (int* pNumbers = numbers)
{
// UNSAFE: Pointer declaration and manipulation
int* p = pNumbers;
// UNSAFE: Dereferencing pointers to modify memory directly
*p = *p + 5;
*(p + 1) = *(p + 1) + 5;
}
}
Why Use Unsafe Code?
There are several legitimate reasons to use unsafe code:
- Performance optimization: For extremely performance-critical sections where you need to eliminate overhead from bounds checking or other safety features.
- Interoperability: When interfacing with native libraries or system APIs that require pointers.
- Low-level operations: For systems programming tasks that require direct memory manipulation, like implementing custom memory managers.
- Hardware access: When working directly with device drivers or memory-mapped hardware.
- Algorithms requiring pointer arithmetic: Some specialized algorithms are most efficiently implemented using pointer operations.
Risks and Considerations
Using unsafe code comes with significant responsibilities:
- You bypass the runtime’s safety checks, so errors can cause application crashes or security vulnerabilities
- Memory leaks are possible if you allocate unmanaged memory and don’t free it properly
- Your code becomes less portable across different .NET implementations
- Debugging unsafe code is more challenging
In general, you should only use unsafe code when absolutely necessary and isolate it in small, well-tested sections of your application.
In conclusion, I’m happy to see that the Uno platform has matured significantly. While there are still some challenges like handling unsafe generated code, the setup process has become much more reliable. If you’re looking to develop truly cross-platform applications with a single codebase, Uno is worth exploring – just remember to uncomment that AllowUnsafeBlocks setting if you run into compilation issues!
by Joche Ojeda | Mar 2, 2025 | C#, System Theory
This past week, I have been working on a prototype for a wizard component. As you might know, in computer interfaces, wizard components (or multi-step forms) allow users to navigate through a finite number of steps or pages until they reach the end. Wizards are particularly useful because they don’t overwhelm users with too many choices at once, effectively minimizing the number of decisions a user needs to make at any specific moment.
The current prototype is created using XAF from DevExpress. If you follow this blog, you probably know that I’m a DevExpress MVP, and I wanted to use their tools to create this prototype.
I’ve built wizard components before, but mostly in a rush. Those previous implementations had the wizard logic hardcoded directly inside the UI components, with no separation between the UI and the underlying logic. While they worked, they were quite messy. This time, I wanted to take a more structured approach to creating a wizard component, so here are a few of my findings. Most of this might seem obvious, but sometimes it’s hard to see the forest for the trees when you’re sitting in front of the computer writing code.
Understanding the Core Concept: State Machines
To create an effective wizard component, you need to understand several underlying concepts. The idea of a wizard is actually rooted in system theory and computer science—it’s essentially an implementation of what’s called a state machine or finite state machine.
Theory of a State Machine
A state machine is the same as a finite state machine (FSM). Both terms refer to a computational model that describes a system existing in one of a finite number of states at any given time.
A state machine (or FSM) consists of:
- States: Distinct conditions the system can be in
- Transitions: Rules for moving between states
- Events/Inputs: Triggers that cause transitions
- Actions: Operations performed when entering/exiting states or during transitions
The term “finite” emphasizes that there’s a limited, countable number of possible states. This finite nature is crucial as it makes the system predictable and analyzable.
State machines come in several variants:
- Deterministic FSMs (one transition per input)
- Non-deterministic FSMs (multiple possible transitions per input)
- Mealy machines (outputs depend on state and input)
- Moore machines (outputs depend only on state)
They’re widely used in software development, hardware design, linguistics, and many other fields because they make complex behavior easier to visualize, implement, and debug. Common examples include traffic lights, UI workflows, network protocols, and parsers.
In practical usage, when someone refers to a “state machine,” they’re almost always talking about a finite state machine.
Implementing a Wizard State Machine
Here’s an implementation of a wizard state machine that separates the logic from the UI:
public class WizardStateMachineBase
{
readonly List<WizardPage> _pages;
int _currentIndex;
public WizardStateMachineBase(IEnumerable<WizardPage> pages)
{
_pages = pages.OrderBy(p => p.Index).ToList();
_currentIndex = 0;
}
public event EventHandler<StateTransitionEventArgs> StateTransition;
public WizardPage CurrentPage => _pages[_currentIndex];
public virtual bool MoveNext()
{
if (_currentIndex < _pages.Count - 1) { var args = new StateTransitionEventArgs(CurrentPage, _pages[_currentIndex + 1]); OnStateTransition(args); if (!args.Cancel) { _currentIndex++; return true; } } return false; } public virtual bool MovePrevious() { if (_currentIndex > 0)
{
var args = new StateTransitionEventArgs(CurrentPage, _pages[_currentIndex - 1]);
OnStateTransition(args);
if (!args.Cancel)
{
_currentIndex--;
return true;
}
}
return false;
}
protected virtual void OnStateTransition(StateTransitionEventArgs e)
{
StateTransition?.Invoke(this, e);
}
}
public class StateTransitionEventArgs : EventArgs
{
public WizardPage CurrentPage { get; }
public WizardPage NextPage { get; }
public bool Cancel { get; set; }
public StateTransitionEventArgs(WizardPage currentPage, WizardPage nextPage)
{
CurrentPage = currentPage;
NextPage = nextPage;
Cancel = false;
}
}
public class WizardPage
{
public int Index { get; set; }
public string Title { get; set; }
public string Description { get; set; }
public bool IsRequired { get; set; } = true;
public bool IsCompleted { get; set; }
// Additional properties specific to your wizard implementation
public object Content { get; set; }
public WizardPage(int index, string title)
{
Index = index;
Title = title;
}
public virtual bool Validate()
{
// Default implementation assumes page is valid
// Override this method in derived classes to provide specific validation logic
return true;
}
}
Benefits of This Approach
As you can see, by defining a state machine, you significantly narrow down the implementation possibilities. You solve the problem of “too many parts to consider” – questions like “How do I start?”, “How do I control the state?”, “Should the state be in the UI or a separate class?”, and so on. These problems can become really complicated, especially if you don’t centralize the state control.
This simple implementation of a wizard state machine shows how to centralize control of the component’s state. By separating the state management from the UI components, we create a cleaner, more maintainable architecture.
The WizardStateMachineBase
class manages the collection of pages and handles navigation between them, while the StateTransitionEventArgs
class provides a mechanism to cancel transitions if needed (for example, if validation fails). The newly added WizardPage
class encapsulates all the information needed for each step in the wizard.
What’s Next?
The next step will be to control how the visual components react to the state of the machine – essentially connecting our state machine to the UI layer. This will include handling the display of the current page content, updating navigation buttons (previous/next/finish), and possibly showing progress indicators. I’ll cover this UI integration in my next post.
By following this pattern, you can create wizard interfaces that are not only user-friendly but also maintainable and extensible from a development perspective.
Source Code
egarim/WizardStateMachineTest
About US
YouTube
https://www.youtube.com/c/JocheOjedaXAFXAMARINC
Our sites
https://www.bitframeworks.com
https://www.xari.io
https://www.xafers.training
Let’s discuss your XAF Support needs together! This 1-hour call/zoom will give you the opportunity to define the roadblocks in your current XAF solution
Schedule a meeting with us on this link
by Joche Ojeda | Mar 2, 2025 | PowerShell, WebServers, Windows Os
In one of our meetings with Javier, we were discussing how many servers we have in the office. In the end, it turned out that we have a lot of servers, both Windows and Linux. So we decided to take a look and see what is running on each of the servers. A lot of those servers are actually test servers used to test deployments or to show something to a customer. A few of them were just full of examples or basically nothing, so I decided to format them and rebuild the installation.
I decided to start with the Windows server, so this post is going to be about the tools that I use to set up the Windows 2016 server.
There were a few tasks that I needed to accomplish that I usually do using the UI, and most of them are a pain in the ****. There is no other way to describe it, so I decided to create scripts instead so I can replicate it easily between servers.
Disable Internet Explorer Enhanced Security
The first task that I do when I set up a Windows Server is to disable Internet Explorer Enhanced Security. If you have used that type of security before, basically it means that you need to allow or whitelist every URL in the page that you’re browsing and the related pages in the page that you’re browsing. So it’s like 100 clicks per page. To remove the enhanced security, you need to go to the Windows features and turn it off there and then restart. So I created a script that does that for me. In one click, I can just disable the security so I can use Internet Explorer to actually download something newer like Microsoft Edge.
Disable internet explorer Enhanced Security
Set Up Web Server Role
The next step after disabling the enhanced security is to set up the Web Server role in Windows Server. This doesn’t come out of the box; you have to actually add the role to the server. For that, I will use another script, and the script will also install Web Deploy, which is the functionality that allows you to do remote deploying into an IIS server.
Setup Web Server Role
Fix Web Deploy Permissions (Optional)
Now here is an extra step. This step is optional. For some reason, in some of the servers, even though you have a clean installation, there will be a problem setting up the Web Deploy functionality. It’s a permission problem basically. So there is a script to fix that. You have to run the first script that installs the Web Server and the remote Web Deploy functionality. This is optional; you should use it only in case your Web Deploy doesn’t work.
Fix web deploy permissions
Set Up SQL Server Express
The next step for setting up the server is to set up SQL Server Express, and I have a script for that. I will paste it here, but for some reason, the script always fails in the way that you download and try to deploy it on the server. What happens is that the process always gets busy and the files get locked. So I will have to come back to that one later, but I will post the script here just to remember it.
SqlServer Express install
Enable Remote SQL Server Access (Optional)
OK, the next script is also optional. In our test servers, we usually allow remote access to the SQL Server database because we need to either restore a backup or create a database. For this, we need to do two things: open the firewall port for the database and also enable TCP connection from the outside. So here is a script for that too.
Enable Remote SQL Server Access
So that will be it for this post. Let me know which script you would like to have to automate your server setup.
by Joche Ojeda | Feb 24, 2025 | Linux, Postgres, SyncFrameworkV2, Ubuntu, WSL
After a problematic Windows update on my Surface computer that prevented me from compiling .NET applications, I spent days trying various fixes without success. Eventually, I had to format my computer and start fresh. This meant setting up everything again – Visual Studio, testing databases, and all the other development tools.To make future setups easier, I created a collection of WSL 2 scripts that automate the installation of tools I frequently use, like PostgreSQL and MySQL for testing purposes. While these scripts contain some practices that wouldn’t be recommended for production (like hardcoded passwords), they’re specifically designed for testing environments. The passwords used are already present in the sync framework source code, so there’s no additional security risk.I decided to share these scripts not as a perfect solution, but as a starting point for others who might need to set up similar testing environments. You can use them as inspiration for your own scripts or modify the default passwords to match your needs.
Note that these are specifically for testing purposes – particularly for working with the sync framework – and the hardcoded credentials should never be used in a production environment.
https://github.com/egarim/MyWslScripts
LDAP Scripts
MyWslScripts/ldap-setup.sh at master · egarim/MyWslScripts
MyWslScripts/add-ldap-user.sh at master · egarim/MyWslScripts
MySQL
MyWslScripts/install_mysql.sh at master · egarim/MyWslScripts
Postgres
MyWslScripts/install_postgres.sh at master · egarim/MyWslScripts
Redis
MyWslScripts/redis-install.sh at master · egarim/MyWslScripts
Let me know if you’d like me to share the actual scripts in a follow-up post!