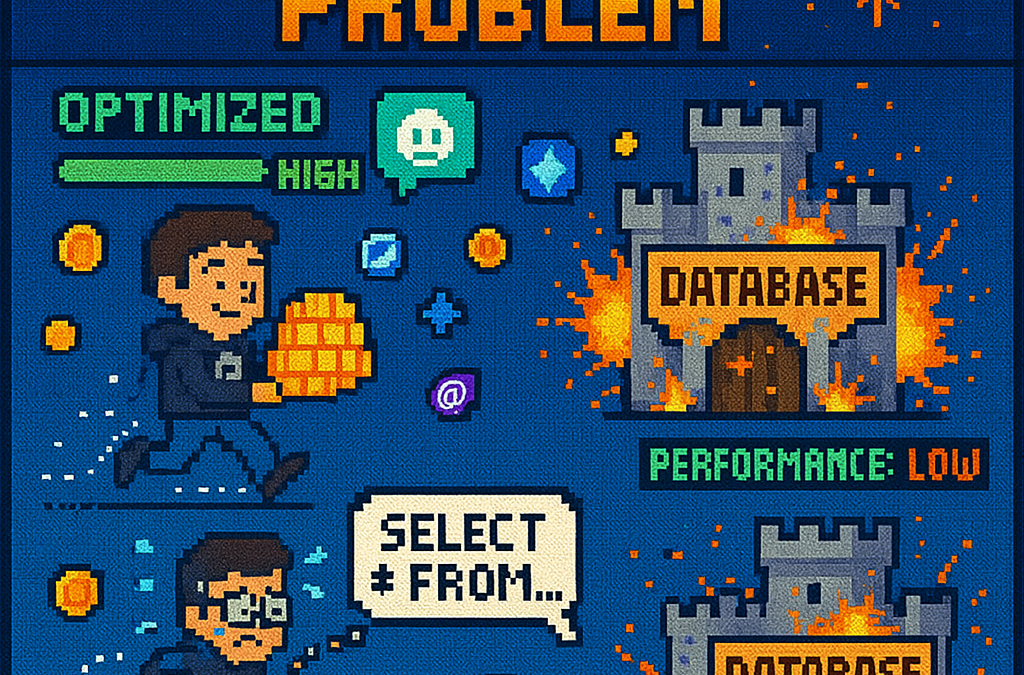
Understanding the N+1 Database Problem using Entity Framework Core
What is the N+1 Problem?
Imagine you’re running a blog website and want to display a list of all blogs along with how many posts each one has. The N+1 problem is a common database performance issue that happens when your application makes way too many database trips to get this simple information.
Our Test Database Setup
Our test suite creates a realistic blog scenario with:
- 3 different blogs
- Multiple posts for each blog
- Comments on posts
- Tags associated with blogs
This mirrors real-world applications where data is interconnected and needs to be loaded efficiently.
Test Case 1: The Classic N+1 Problem (Lazy Loading)
What it does: This test demonstrates how “lazy loading” can accidentally create the N+1 problem. Lazy loading sounds helpful – it automatically fetches related data when you need it. But this convenience comes with a hidden cost.
The Code:
[Test]
public void Test_N_Plus_One_Problem_With_Lazy_Loading()
{
var blogs = _context.Blogs.ToList(); // Query 1: Load blogs
foreach (var blog in blogs)
{
var postCount = blog.Posts.Count; // Each access triggers a query!
TestLogger.WriteLine($"Blog: {blog.Title} - Posts: {postCount}");
}
}
The SQL Queries Generated:
-- Query 1: Load all blogs
SELECT "b"."Id", "b"."CreatedDate", "b"."Description", "b"."Title"
FROM "Blogs" AS "b"
-- Query 2: Load posts for Blog 1 (triggered by lazy loading)
SELECT "p"."Id", "p"."BlogId", "p"."Content", "p"."PublishedDate", "p"."Title"
FROM "Posts" AS "p"
WHERE "p"."BlogId" = 1
-- Query 3: Load posts for Blog 2 (triggered by lazy loading)
SELECT "p"."Id", "p"."BlogId", "p"."Content", "p"."PublishedDate", "p"."Title"
FROM "Posts" AS "p"
WHERE "p"."BlogId" = 2
-- Query 4: Load posts for Blog 3 (triggered by lazy loading)
SELECT "p"."Id", "p"."BlogId", "p"."Content", "p"."PublishedDate", "p"."Title"
FROM "Posts" AS "p"
WHERE "p"."BlogId" = 3
The Problem: 4 total queries (1 + 3) – Each time you access blog.Posts.Count
, lazy loading triggers a separate database trip.
Test Case 2: Alternative N+1 Demonstration
What it does: This test manually recreates the N+1 pattern to show exactly what’s happening, even if lazy loading isn’t working properly.
The Code:
[Test]
public void Test_N_Plus_One_Problem_Alternative_Approach()
{
var blogs = _context.Blogs.ToList(); // Query 1
foreach (var blog in blogs)
{
// This explicitly loads posts for THIS blog only (simulates lazy loading)
var posts = _context.Posts.Where(p => p.BlogId == blog.Id).ToList();
TestLogger.WriteLine($"Loaded {posts.Count} posts for blog {blog.Id}");
}
}
The Lesson: This explicitly demonstrates the N+1 pattern with manual queries. The result is identical to lazy loading – one query per blog plus the initial blogs query.
Test Case 3: N+1 vs Include() – Side by Side Comparison
What it does: This is the money shot – a direct comparison showing the dramatic difference between the problematic approach and the solution.
The Bad Code (N+1):
// BAD: N+1 Problem
var blogsN1 = _context.Blogs.ToList(); // Query 1
foreach (var blog in blogsN1)
{
var posts = _context.Posts.Where(p => p.BlogId == blog.Id).ToList(); // Queries 2,3,4...
}
The Good Code (Include):
// GOOD: Include() Solution
var blogsInclude = _context.Blogs
.Include(b => b.Posts)
.ToList(); // Single query with JOIN
foreach (var blog in blogsInclude)
{
// No additional queries needed - data is already loaded!
var postCount = blog.Posts.Count;
}
The SQL Queries:
Bad Approach (Multiple Queries):
-- Same 4 separate queries as shown in Test Case 1
Good Approach (Single Query):
SELECT "b"."Id", "b"."CreatedDate", "b"."Description", "b"."Title",
"p"."Id", "p"."BlogId", "p"."Content", "p"."PublishedDate", "p"."Title"
FROM "Blogs" AS "b"
LEFT JOIN "Posts" AS "p" ON "b"."Id" = "p"."BlogId"
ORDER BY "b"."Id"
Results from our test:
- Bad approach: 4 total queries (1 + 3)
- Good approach: 1 total query
- Performance improvement: 75% fewer database round trips!
Test Case 4: Guaranteed N+1 Problem
What it does: This test removes any doubt by explicitly demonstrating the N+1 pattern with clear step-by-step output.
The Code:
[Test]
public void Test_Guaranteed_N_Plus_One_Problem()
{
var blogs = _context.Blogs.ToList(); // Query 1
int queryCount = 1;
foreach (var blog in blogs)
{
queryCount++;
// This explicitly demonstrates the N+1 pattern
var posts = _context.Posts.Where(p => p.BlogId == blog.Id).ToList();
TestLogger.WriteLine($"Loading posts for blog '{blog.Title}' (Query #{queryCount})");
}
}
Why it’s useful: This ensures we can always see the problem clearly by manually executing the problematic pattern, making it impossible to miss.
Test Case 5: Eager Loading with Include()
What it does: Shows the correct way to load related data upfront using Include()
.
The Code:
[Test]
public void Test_Eager_Loading_With_Include()
{
var blogsWithPosts = _context.Blogs
.Include(b => b.Posts)
.ToList();
foreach (var blog in blogsWithPosts)
{
// No additional queries - data already loaded!
TestLogger.WriteLine($"Blog: {blog.Title} - Posts: {blog.Posts.Count}");
}
}
The SQL Query:
SELECT "b"."Id", "b"."CreatedDate", "b"."Description", "b"."Title",
"p"."Id", "p"."BlogId", "p"."Content", "p"."PublishedDate", "p"."Title"
FROM "Blogs" AS "b"
LEFT JOIN "Posts" AS "p" ON "b"."Id" = "p"."BlogId"
ORDER BY "b"."Id"
The Benefit: One database trip loads everything. When you access blog.Posts.Count
, the data is already there.
Test Case 6: Multiple Includes with ThenInclude()
What it does: Demonstrates loading deeply nested data – blogs → posts → comments – all in one query.
The Code:
[Test]
public void Test_Multiple_Includes_With_ThenInclude()
{
var blogsWithPostsAndComments = _context.Blogs
.Include(b => b.Posts)
.ThenInclude(p => p.Comments)
.ToList();
foreach (var blog in blogsWithPostsAndComments)
{
foreach (var post in blog.Posts)
{
// All data loaded in one query!
TestLogger.WriteLine($"Post: {post.Title} - Comments: {post.Comments.Count}");
}
}
}
The SQL Query:
SELECT "b"."Id", "b"."CreatedDate", "b"."Description", "b"."Title",
"p"."Id", "p"."BlogId", "p"."Content", "p"."PublishedDate", "p"."Title",
"c"."Id", "c"."Author", "c"."Content", "c"."CreatedDate", "c"."PostId"
FROM "Blogs" AS "b"
LEFT JOIN "Posts" AS "p" ON "b"."Id" = "p"."BlogId"
LEFT JOIN "Comments" AS "c" ON "p"."Id" = "c"."PostId"
ORDER BY "b"."Id", "p"."Id"
The Challenge: Loading three levels of data in one optimized query instead of potentially hundreds of separate queries.
Test Case 7: Projection with Select()
What it does: Shows how to load only the specific data you actually need instead of entire objects.
The Code:
[Test]
public void Test_Projection_With_Select()
{
var blogData = _context.Blogs
.Select(b => new
{
BlogTitle = b.Title,
PostCount = b.Posts.Count(),
RecentPosts = b.Posts
.OrderByDescending(p => p.PublishedDate)
.Take(2)
.Select(p => new { p.Title, p.PublishedDate })
})
.ToList();
}
The SQL Query (from our test output):
SELECT "b"."Title", (
SELECT COUNT(*)
FROM "Posts" AS "p"
WHERE "b"."Id" = "p"."BlogId"), "b"."Id", "t0"."Title", "t0"."PublishedDate", "t0"."Id"
FROM "Blogs" AS "b"
LEFT JOIN (
SELECT "t"."Title", "t"."PublishedDate", "t"."Id", "t"."BlogId"
FROM (
SELECT "p0"."Title", "p0"."PublishedDate", "p0"."Id", "p0"."BlogId",
ROW_NUMBER() OVER(PARTITION BY "p0"."BlogId" ORDER BY "p0"."PublishedDate" DESC) AS "row"
FROM "Posts" AS "p0"
) AS "t"
WHERE "t"."row" <= 2
) AS "t0" ON "b"."Id" = "t0"."BlogId"
ORDER BY "b"."Id", "t0"."BlogId", "t0"."PublishedDate" DESC
Why it matters: This query only loads the specific fields needed, uses window functions for efficiency, and calculates counts in the database rather than loading full objects.
Test Case 8: Split Query Strategy
What it does: Demonstrates an alternative approach where one large JOIN is split into multiple optimized queries.
The Code:
[Test]
public void Test_Split_Query()
{
var blogs = _context.Blogs
.AsSplitQuery()
.Include(b => b.Posts)
.Include(b => b.Tags)
.ToList();
}
The SQL Queries (from our test output):
-- Query 1: Load blogs
SELECT "b"."Id", "b"."CreatedDate", "b"."Description", "b"."Title"
FROM "Blogs" AS "b"
ORDER BY "b"."Id"
-- Query 2: Load posts (automatically generated)
SELECT "p"."Id", "p"."BlogId", "p"."Content", "p"."PublishedDate", "p"."Title", "b"."Id"
FROM "Blogs" AS "b"
INNER JOIN "Posts" AS "p" ON "b"."Id" = "p"."BlogId"
ORDER BY "b"."Id"
-- Query 3: Load tags (automatically generated)
SELECT "t"."Id", "t"."Name", "b"."Id"
FROM "Blogs" AS "b"
INNER JOIN "BlogTag" AS "bt" ON "b"."Id" = "bt"."BlogsId"
INNER JOIN "Tags" AS "t" ON "bt"."TagsId" = "t"."Id"
ORDER BY "b"."Id"
When to use it: When JOINing lots of related data creates one massive, slow query. Split queries break this into several smaller, faster queries.
Test Case 9: Filtered Include()
What it does: Shows how to load only specific related data – in this case, only recent posts from the last 15 days.
The Code:
[Test]
public void Test_Filtered_Include()
{
var cutoffDate = DateTime.Now.AddDays(-15);
var blogsWithRecentPosts = _context.Blogs
.Include(b => b.Posts.Where(p => p.PublishedDate > cutoffDate))
.ToList();
}
The SQL Query:
SELECT "b"."Id", "b"."CreatedDate", "b"."Description", "b"."Title",
"p"."Id", "p"."BlogId", "p"."Content", "p"."PublishedDate", "p"."Title"
FROM "Blogs" AS "b"
LEFT JOIN "Posts" AS "p" ON "b"."Id" = "p"."BlogId" AND "p"."PublishedDate" > @cutoffDate
ORDER BY "b"."Id"
The Efficiency: Only loads posts that meet the criteria, reducing data transfer and memory usage.
Test Case 10: Explicit Loading
What it does: Demonstrates manually controlling when related data gets loaded.
The Code:
[Test]
public void Test_Explicit_Loading()
{
var blogs = _context.Blogs.ToList(); // Load blogs only
// Now explicitly load posts for all blogs
foreach (var blog in blogs)
{
_context.Entry(blog)
.Collection(b => b.Posts)
.Load();
}
}
The SQL Queries:
-- Query 1: Load blogs
SELECT "b"."Id", "b"."CreatedDate", "b"."Description", "b"."Title"
FROM "Blogs" AS "b"
-- Query 2: Explicitly load posts for blog 1
SELECT "p"."Id", "p"."BlogId", "p"."Content", "p"."PublishedDate", "p"."Title"
FROM "Posts" AS "p"
WHERE "p"."BlogId" = 1
-- Query 3: Explicitly load posts for blog 2
SELECT "p"."Id", "p"."BlogId", "p"."Content", "p"."PublishedDate", "p"."Title"
FROM "Posts" AS "p"
WHERE "p"."BlogId" = 2
-- ... and so on
When useful: When you sometimes need related data and sometimes don’t. You control exactly when the additional database trip happens.
Test Case 11: Batch Loading Pattern
What it does: Shows a clever technique to avoid N+1 by loading all related data in one query, then organizing it in memory.
The Code:
[Test]
public void Test_Batch_Loading_Pattern()
{
var blogs = _context.Blogs.ToList(); // Query 1
var blogIds = blogs.Select(b => b.Id).ToList();
// Single query to get all posts for all blogs
var posts = _context.Posts
.Where(p => blogIds.Contains(p.BlogId))
.ToList(); // Query 2
// Group posts by blog in memory
var postsByBlog = posts.GroupBy(p => p.BlogId).ToDictionary(g => g.Key, g => g.ToList());
}
The SQL Queries:
-- Query 1: Load all blogs
SELECT "b"."Id", "b"."CreatedDate", "b"."Description", "b"."Title"
FROM "Blogs" AS "b"
-- Query 2: Load ALL posts for ALL blogs in one query
SELECT "p"."Id", "p"."BlogId", "p"."Content", "p"."PublishedDate", "p"."Title"
FROM "Posts" AS "p"
WHERE "p"."BlogId" IN (1, 2, 3)
The Result: Just 2 queries total, regardless of how many blogs you have. Data organization happens in memory.
Test Case 12: Performance Comparison
What it does: Puts all the approaches head-to-head to show their relative performance.
The Code:
[Test]
public void Test_Performance_Comparison()
{
// N+1 Problem (Multiple Queries)
var blogs1 = _context.Blogs.ToList();
foreach (var blog in blogs1)
{
var count = blog.Posts.Count(); // Triggers separate query
}
// Eager Loading (Single Query)
var blogs2 = _context.Blogs
.Include(b => b.Posts)
.ToList();
// Projection (Minimal Data)
var blogSummaries = _context.Blogs
.Select(b => new { b.Title, PostCount = b.Posts.Count() })
.ToList();
}
The SQL Queries Generated:
N+1 Problem: 4 separate queries (as shown in previous examples)
Eager Loading: 1 JOIN query (as shown in Test Case 5)
Projection: 1 optimized query with subquery:
SELECT "b"."Title", (
SELECT COUNT(*)
FROM "Posts" AS "p"
WHERE "b"."Id" = "p"."BlogId") AS "PostCount"
FROM "Blogs" AS "b"
Real-World Performance Impact
Let’s scale this up to see why it matters:
Small Application (10 blogs):
- N+1 approach: 11 queries (≈110ms)
- Optimized approach: 1 query (≈10ms)
- Time saved: 100ms
Medium Application (100 blogs):
- N+1 approach: 101 queries (≈1,010ms)
- Optimized approach: 1 query (≈10ms)
- Time saved: 1 second
Large Application (1000 blogs):
- N+1 approach: 1001 queries (≈10,010ms)
- Optimized approach: 1 query (≈10ms)
- Time saved: 10 seconds
Key Takeaways
- The N+1 problem gets exponentially worse as your data grows
- Lazy loading is convenient but dangerous – it can hide performance problems
- Include() is your friend for loading related data efficiently
- Projection is powerful when you only need specific fields
- Different problems need different solutions – there’s no one-size-fits-all approach
- SQL query inspection is crucial – always check what queries your ORM generates
The Bottom Line
This test suite shows that small changes in how you write database queries can transform a slow, database-heavy operation into a fast, efficient one. The difference between a frustrated user waiting 10 seconds for a page to load and a happy user getting instant results often comes down to understanding and avoiding the N+1 problem.
The beauty of these tests is that they use real database queries with actual SQL output, so you can see exactly what’s happening under the hood. Understanding these patterns will make you a more effective developer and help you build applications that stay fast as they grow.
You can find the source for this article in my here