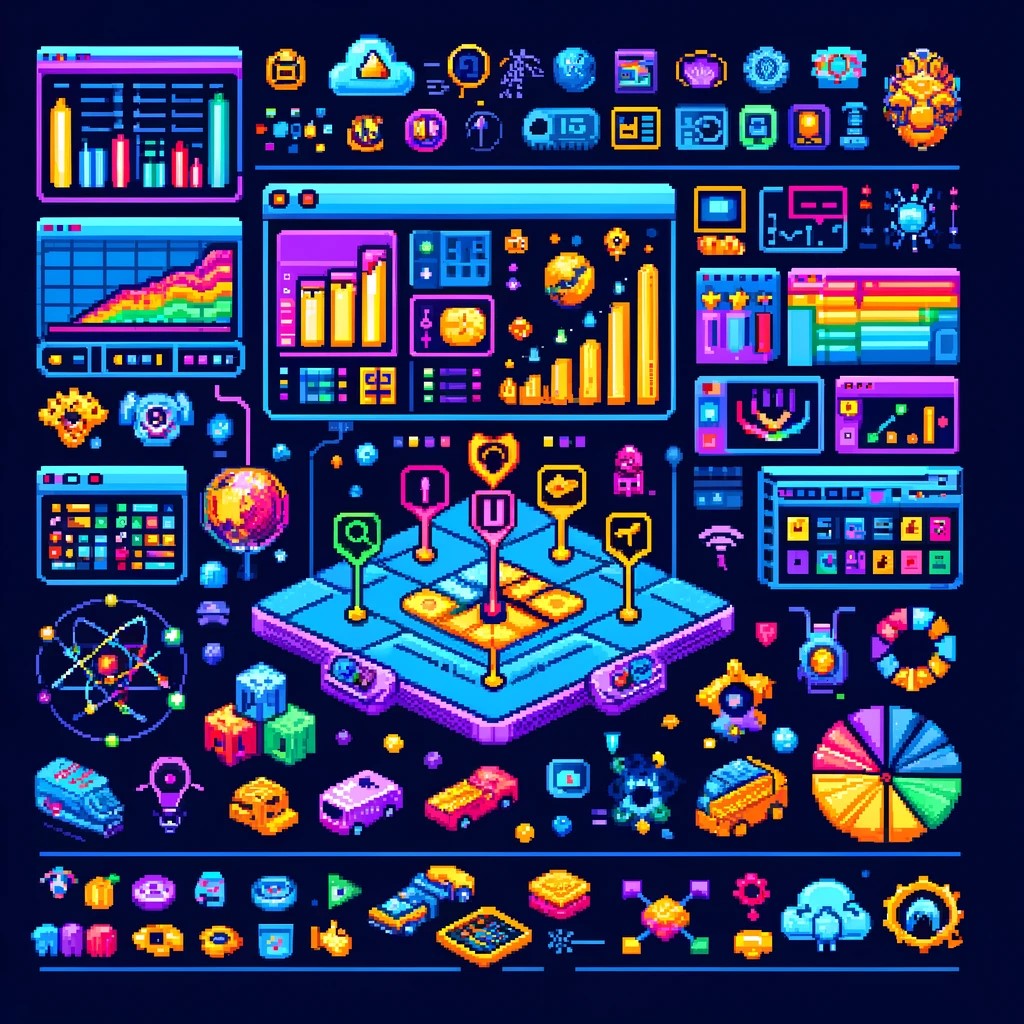
Unlocking the Power of Augmented Data Models: Enhance Analytics and AI Integration for Better Insights
In today’s data-driven world, the need for more sophisticated and insightful data models has never been greater. Traditional database models, while powerful, often fall short of delivering the depth and breadth of insights required by modern organizations. Enter the augmented data model, a revolutionary approach that extends beyond the limitations of traditional models by integrating additional data sources, enhanced data features, advanced analytical capabilities, and AI-driven techniques. This blog post explores the key components, applications, and benefits of augmented data models.
Key Components of an Augmented Data Model
1. Integration of Diverse Data Sources
An augmented data model combines structured, semi-structured, and unstructured data from various sources such as databases, data lakes, social media, IoT devices, and external data feeds. This integration enables a holistic view of data across the organization, breaking down silos and fostering a more interconnected understanding of the data landscape.
2. Enhanced Data Features
Beyond raw data, augmented data models include derived attributes, calculated fields, and metadata to enrich the data. Machine learning and artificial intelligence are employed to create predictive and prescriptive data features, transforming raw data into actionable insights.
3. Advanced Analytics
Augmented data models incorporate advanced analytical models, including machine learning, statistical models, and data mining techniques. These models support real-time analytics and streaming data processing, enabling organizations to make faster, data-driven decisions.
4. AI-Driven Embeddings
One of the standout features of augmented data models is the creation of embeddings. These are dense vector representations of data (such as words, images, or user behaviors) that capture their semantic meaning. Embeddings enhance machine learning models, making them more effective at tasks such as recommendation, natural language processing, and image recognition.
5. Data Visualization and Reporting
To make complex data insights accessible, augmented data models facilitate advanced data visualization tools and dashboards. These tools allow users to interact with data dynamically through self-service analytics platforms, turning data into easily digestible visual stories.
6. Improved Data Quality and Governance
Ensuring data quality is paramount in augmented data models. Automated data cleansing, validation, and enrichment processes maintain high standards of data quality. Robust data governance policies manage data lineage, security, and compliance, ensuring that data is trustworthy and reliable.
7. Scalability and Performance
Designed to handle large volumes of data, augmented data models scale horizontally across distributed systems. They are optimized for high performance in data processing and querying, ensuring that insights are delivered swiftly and efficiently.
Applications and Benefits
Enhanced Decision Making
With deeper insights and predictive capabilities, augmented data models significantly improve decision-making processes. Organizations can move from reactive to proactive strategies, leveraging data to anticipate trends and identify opportunities.
Operational Efficiency
By streamlining data processing and integration, augmented data models reduce manual efforts and errors. This leads to more efficient operations and a greater focus on strategic initiatives.
Customer Insights
Augmented data models enable a 360-degree view of customers by integrating various touchpoints and interactions. This comprehensive view allows for more personalized and effective customer engagement strategies.
Innovation
Supporting advanced analytics and machine learning initiatives, augmented data models foster innovation within the organization. They provide the tools and insights needed to develop new products, services, and business models.
Real-World Examples
Customer 360 Platforms
By combining CRM data, social media interactions, and transactional data, augmented data models create a comprehensive view of customer behavior. This holistic approach enables personalized marketing and improved customer service.
IoT Analytics
Integrating sensor data, machine logs, and external environmental data, augmented data models optimize operations in manufacturing or smart cities. They enable real-time monitoring and predictive maintenance, reducing downtime and increasing efficiency.
Fraud Detection Systems
Using transactional data, user behavior analytics, and external threat intelligence, augmented data models detect and prevent fraudulent activities. Advanced machine learning models identify patterns and anomalies indicative of fraud, providing a proactive defense mechanism.
AI-Powered Recommendations
Embeddings created from user interactions, product descriptions, and historical purchase data power personalized recommendations in e-commerce. These AI-driven insights enhance customer experience and drive sales.
Conclusion
Augmented data models represent a significant advancement in the way organizations handle and analyze data. By leveraging modern technologies and methodologies, including the creation of embeddings for AI, these models provide a more comprehensive and actionable view of the data. The result is enhanced decision-making, improved operational efficiency, deeper customer insights, and a platform for innovation. As organizations continue to navigate the complexities of the data landscape, augmented data models will undoubtedly play a pivotal role in shaping the future of data analytics.