by Joche Ojeda | Apr 2, 2025 | Uncategorized
It’s been a week since the Microsoft MVP Summit, and now I finally sit at Javier’s home trying to write about my trip and experience there. So let’s start!
The Journey
First, I needed to fly via Istanbul. That meant waking up around 2:00 AM to go to the airport and catch my flight at 6:00 AM. In Istanbul, I was really lucky because I was in the new airport which is huge and it has a great business lounge to wait in, so I could get some rest between my flights from Istanbul to Seattle.
I tried to sleep a little. The main problem was that the business lounge was on one side of the airport and my gate was on the other side, about 1 kilometer away. It’s a really big airport! I had to walk all that distance, and they announced the gate really late, so I only had about 15 minutes to get there—a really short time.
After that, I took my flight to the States, from Istanbul to Seattle. The route goes through the Arctic (near the North Pole)—you go up and then a little bit to the right, and then you end up in Seattle. It was a strange route; I’d never used it before. The flight was long, around 15 hours, but it wasn’t bad. I enjoyed Turkish Airlines when they use the big airplanes.
Arrival Challenges
I landed in Seattle around 6:00 PM. Then I had to go through immigration control and collect my luggage, which took almost two hours. After that, I went to the Airbnb, which was super beautiful, but I couldn’t get in because the owners had left the gate closed from the inside, and there were no lights at all, so it was impossible to enter. I waited for two hours for Javier to contact them, and after a while, it started raining, so I decided to go to a hotel. I booked a hotel for the night and took a 30-minute taxi ride. I finally went to bed on Monday at 11:00 PM, which was really late.
Day One at the Summit
The next day, I needed to drop my bags at the Airbnb and go to the MVP Summit. It was a nice experience. Javier was flying in that day and arrived around 3:00 PM, so I went to the first part alone. I missed the keynote because I had to drop off my bags and do all that stuff, so I ended up arriving around 11:00 AM.
The first person I met was Veronica, and we talked for a bit. Then I went to one of the sessions—of course, it was a Copilot session. In the afternoon, I met up with Javier, we grabbed some swag, and went to the Hub. Then I met Pablo from Argentina, and by the end of the day, I got together with Michael Washington, who I always hang out with during the MVP Summits.
Time to go home—it was a long day. We went back to the Airbnb, but didn’t do much. We just watched a TV show that our friend Hector recommended on Netflix.
Day Two: Meeting Peers
For day two, the sessions were great, but what I recall most are my meetings with specific people. When you go to the MVP Summits, you get to meet your peers. Usually, it’s like you’re good at one thing—for example, Javier and I do AI courses, and most of what we write about is general development—but there are people who really specialize.
For instance, I met the people from the Uno team, amazing people. Jerome and his team are always on the bleeding edge of .NET. We talked about the “black magic” they’ve written for their multi-target single application for Uno. It’s always nice to meet the Uno team.
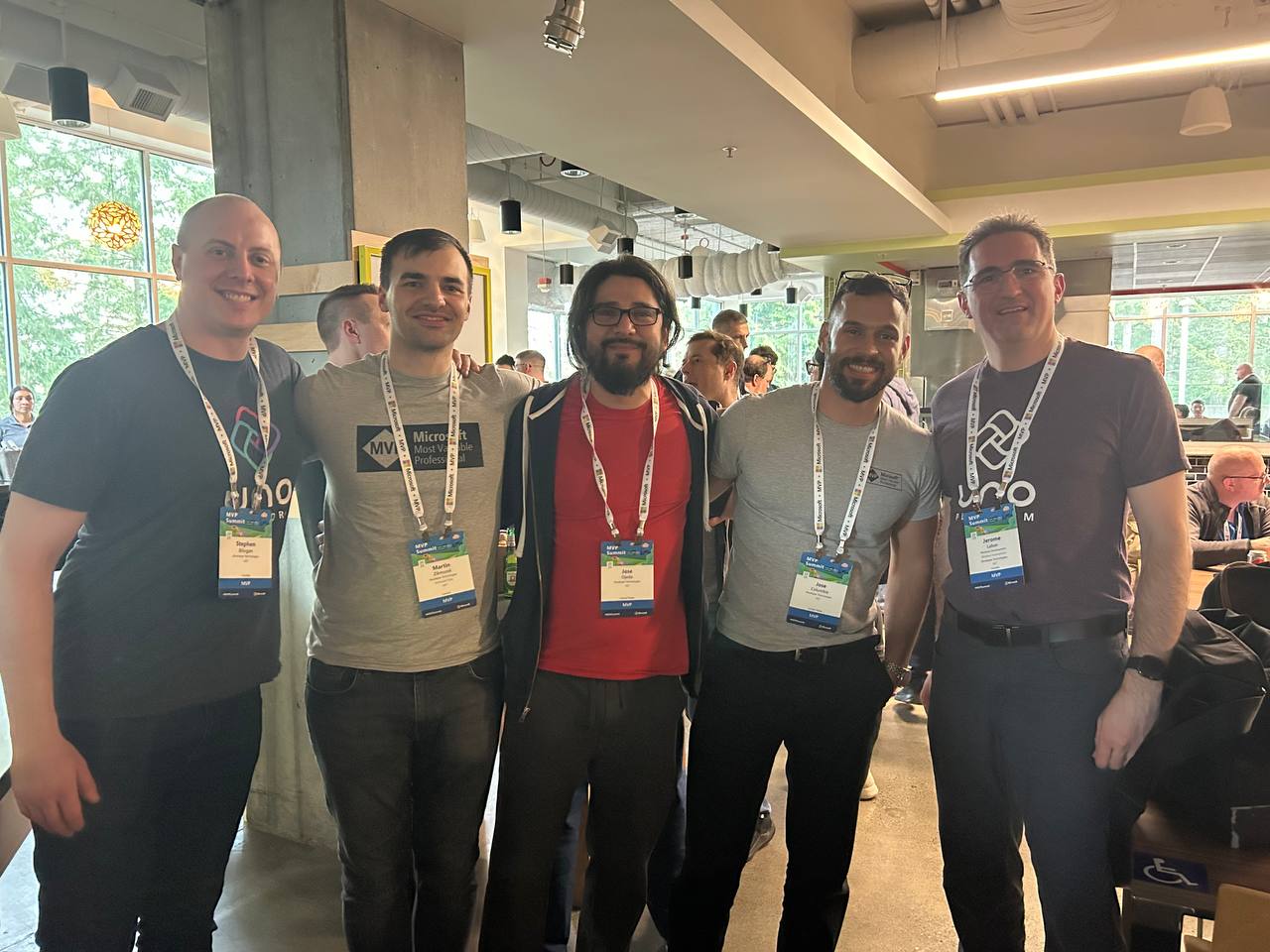
I met with Michael Washington again several times in the hallways of Microsoft, and we talked about how to redirect Microsoft AI extensions to use LLM Studio, which is kind of tricky. It’s not something you can do really easily, like with Semantic Kernel where you only need to replace the HTTP client and then you’re good to go. In LLM Studio, it’s a different trick, so I’ll write about it later.
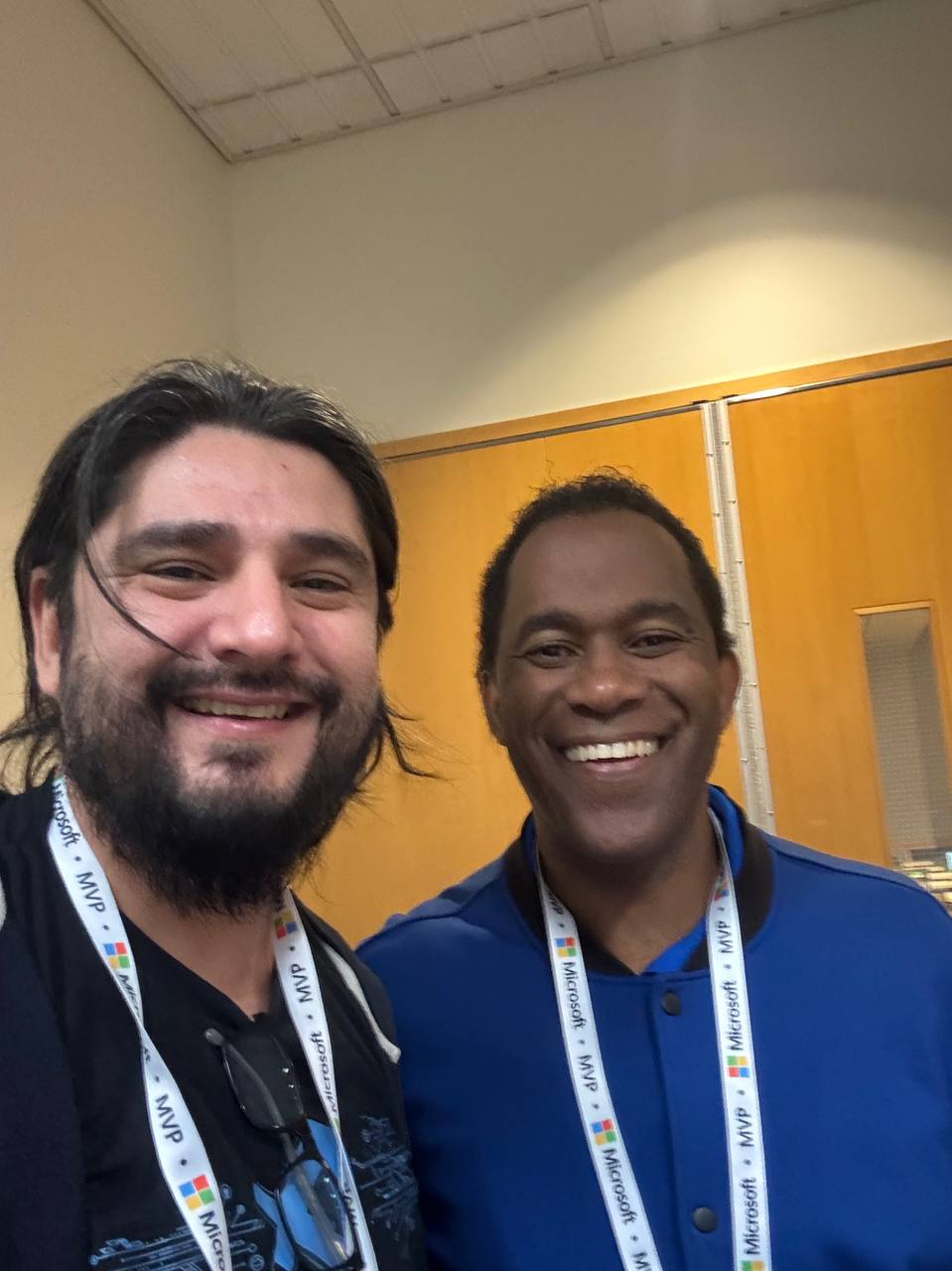
In one of the sessions, Mads Kristensen sat by my side, and I was trying to get some information from him on how to create an extension. Long ago, there was an extension from Oliver Sturm called “Instant Program Gratification” or something similar that displayed a huge congratulation message on the screen every time your compile succeeded, and if it failed, it would display something like “Hey, you need more coffee!” on the screen. I asked Mads how to achieve that with the new extension toolkit, and he explained it to me—he’s the king of extensions for Visual Studio.
Then I met someone new, Jeremy Sinclair, whom Javier introduced me to. We had one of those deep technical conversations about how Windows runs on ARM CPUs and the problems this can bring or how easy some things can be. It’s ironic because the Android architecture is usually ARM, but it doesn’t run on ARM computers because ARM computers emulate x64. We talked a lot about the challenges you might encounter and how to address them. Jeremy has managed to do it; he’s written some articles about what to expect when moving to an ARM computer. He also talked about how the future and the present for MAUI is at the moment.
He was also wearing the Ray-Ban Meta glasses, and I asked him, “Hey, how are they?” He told me they’re nice, though the battery life isn’t great, but they’re kind of fun. So I ordered a pair of Meta Ray-Ban AI glasses, and I like them so far.
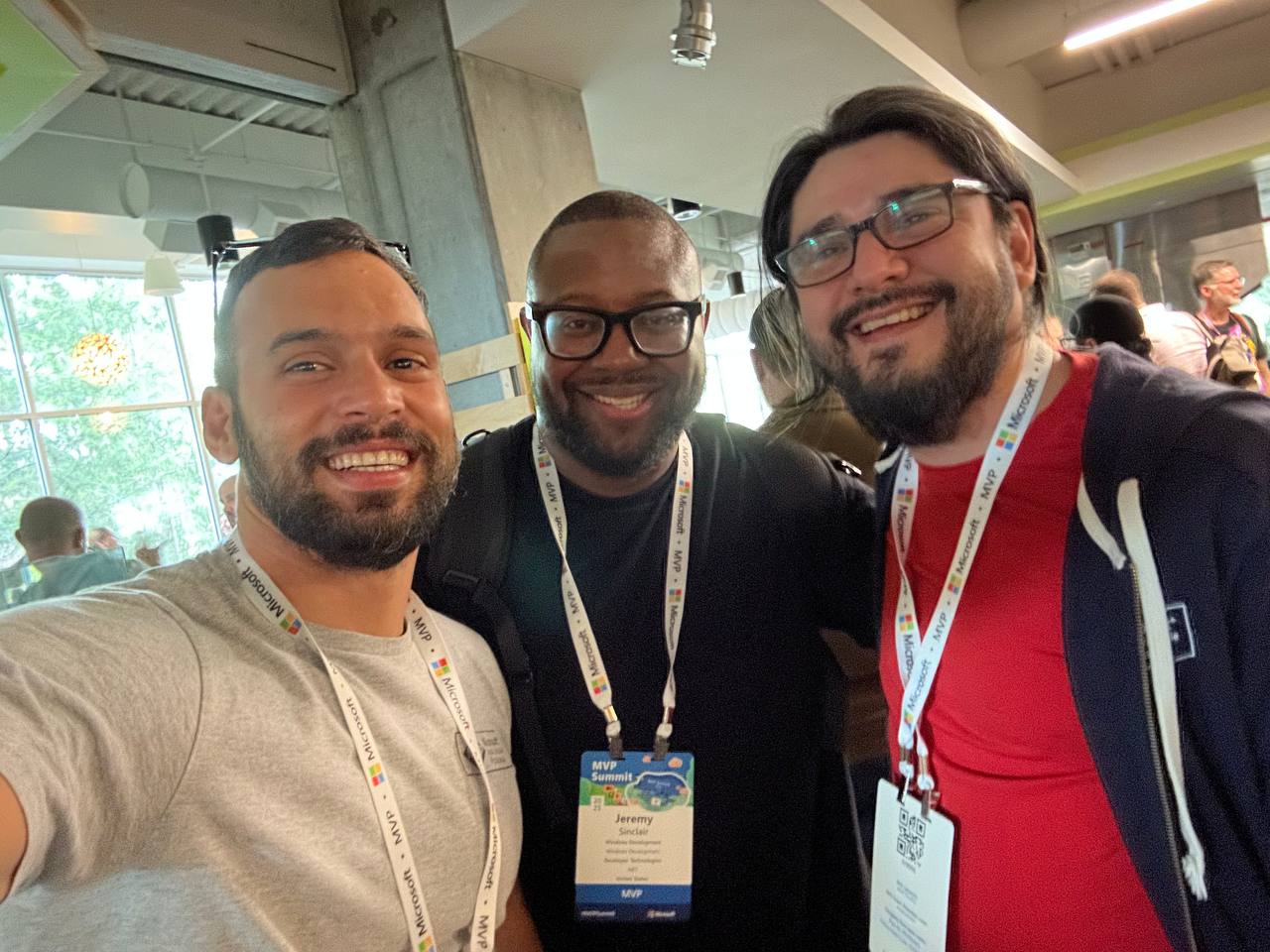
More Memorable Conversations
Another great conversation that we had with Javier was with James Montemagno. We met him in the Hub, and then we talked a lot about how we started. I’ve been a long-term fan of Merge Conflict, their podcast, and Javier introduced me to that podcast a long time ago when we met around 9 years ago. When he was traveling to work, he called me, we talked mostly about development for about one hour on his way to work, and then he told me, “Hey, I listen to this and this podcast, I listen to that and that podcast.” So I became a follower of Merge Conflict after that.
James explained all the adventures on the Xamarin team, how it went when Xamarin joined Microsoft, about the difference between Xamarin from Microsoft and Xamarin from Xamarin Forms, and how life is changing for him as more of a project manager than an advocate. So he’s kind of busy all the time, but we had this really long conversation, like 40 minutes or so. He was really open about talking about his adventure of joining Microsoft and eventually working in the MAUI team.
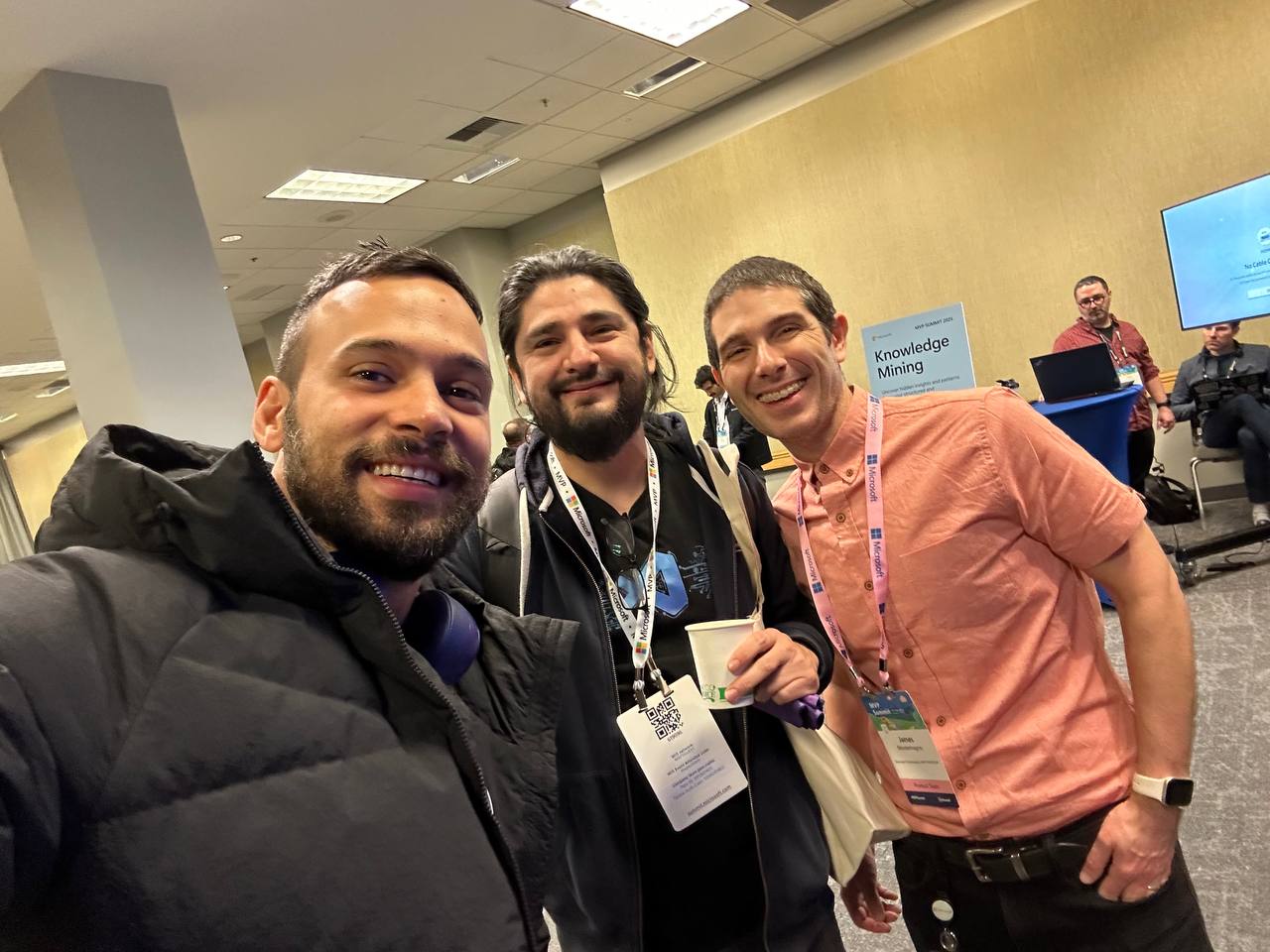
We also met David from the MAUI team, and he was so nice. Long time ago, he featured our company in the list of companies that have made apps with MAUI, and we were on the list they showed in one of the conferences. So we thanked him for that.
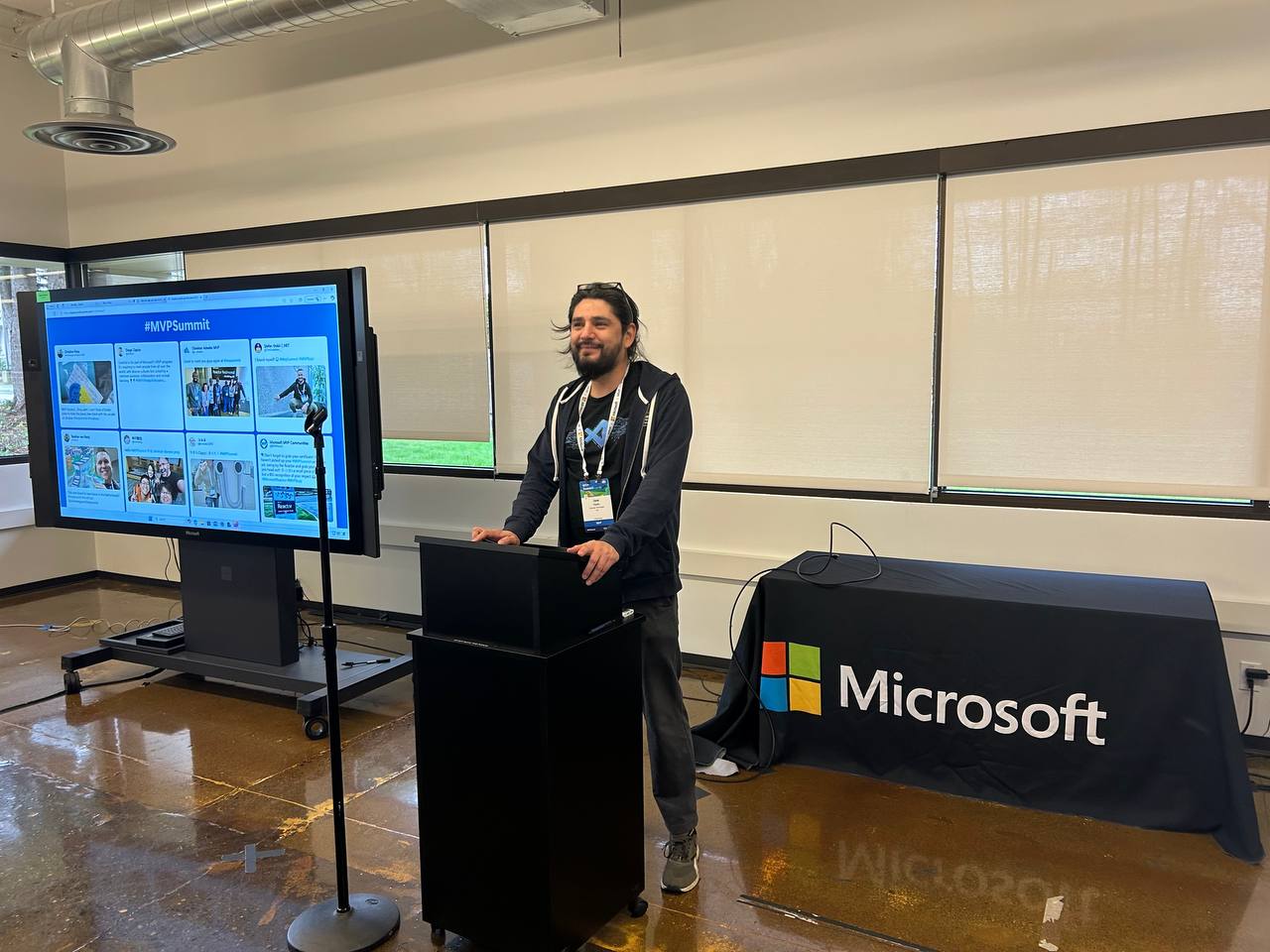
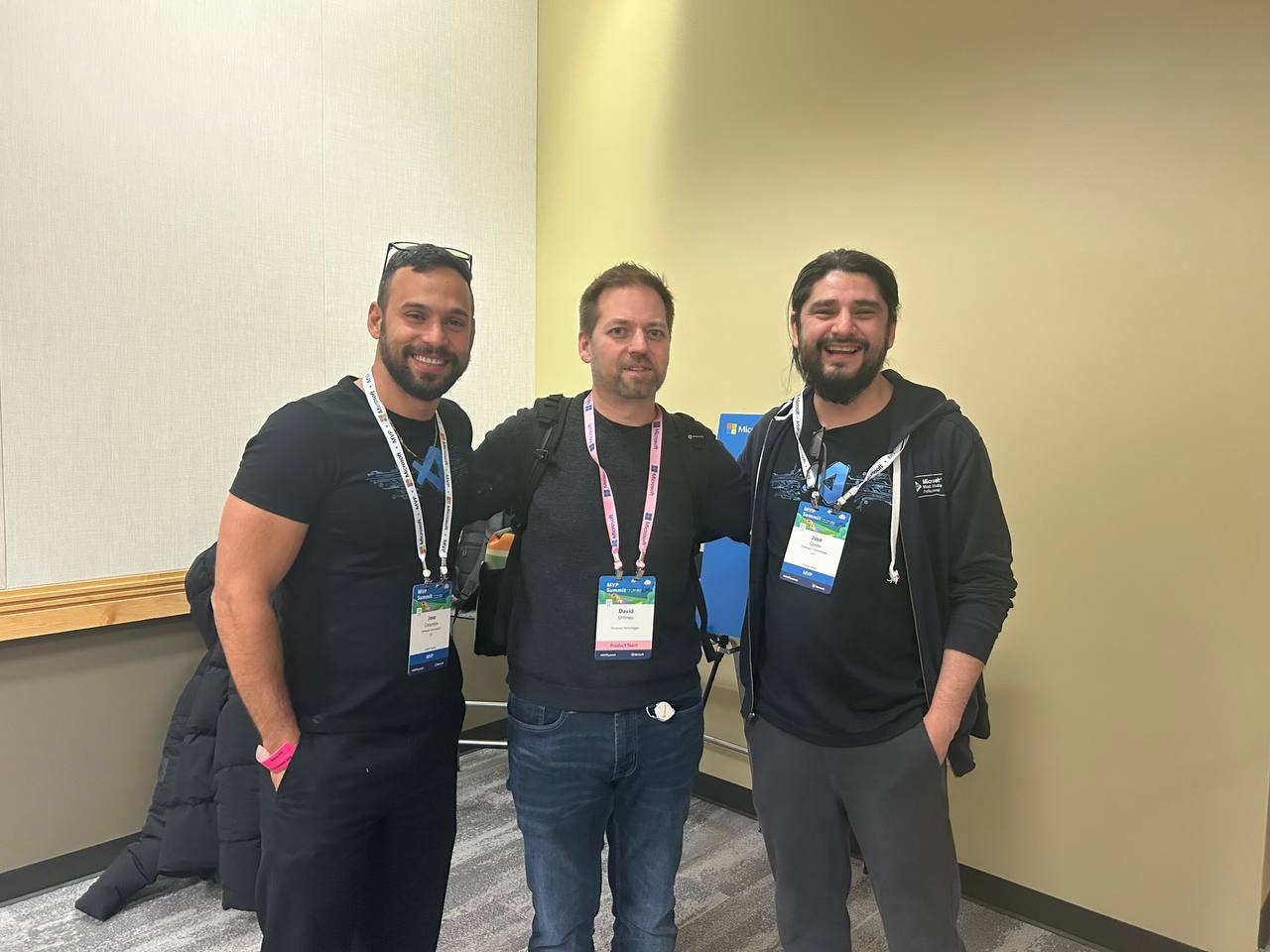
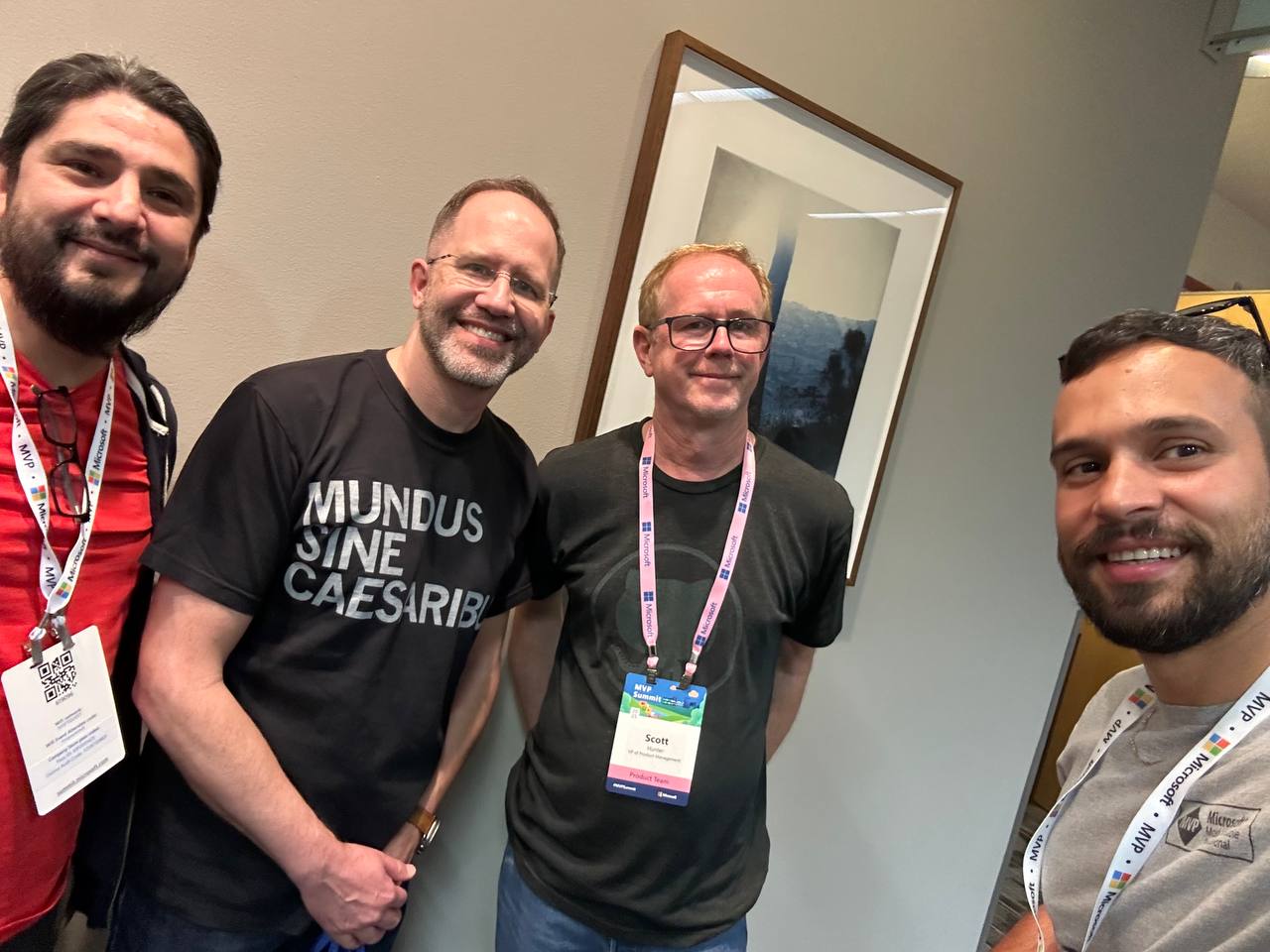
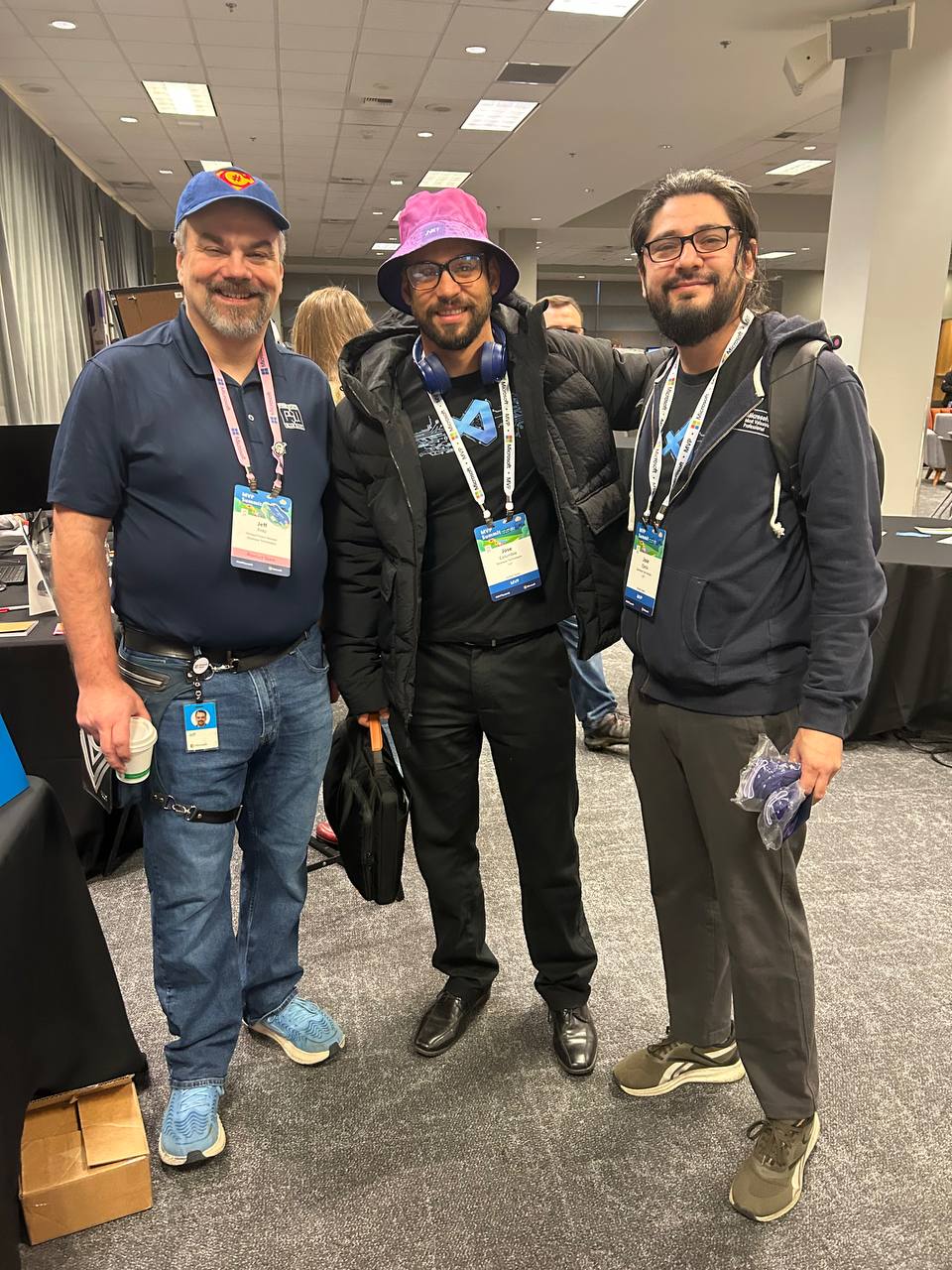
That’s everyone I met at the MVP Summit. I had a great time, and I can’t believe it’s been a year already. I’m looking forward to meeting everyone next year and seeing what we come up with during 2025!
by Joche Ojeda | Nov 2, 2024 | A.I, Semantic Kernel
Today, when I woke up, it was sunny but really cold, and the weather forecast said that snow was expected.
So, I decided to order ramen and do a “Saturday at home” type of project. My tools of choice for this experiment are:
1) DevExpress Chat Component for Blazor
I’m thrilled they have this component. I once wrote my own chat component, and it’s a challenging task, especially given the variety of use cases.
2) Semantic Kernel
I’ve been experimenting with Semantic Kernel for a while now, and let me tell you—it’s a fantastic tool if you’re in the .NET ecosystem. It’s so cool to have native C# code to interact with AI services in a flexible way, making your code mostly agnostic to the AI provider—like a WCF for AIs.
Goal of the Experiment
The goal for today’s experiment is to render a list of products as a carousel within a chat conversation.
Configuration
To accomplish this, I’ll use prompt execution settings in Semantic Kernel to ensure that the response from the LLM is always in JSON format as a string.
var Settings = new OpenAIPromptExecutionSettings
{
MaxTokens = 500,
Temperature = 0.5,
ResponseFormat = "json_object"
};
The key part here is the response format. The chat completion can respond in two ways:
- Text: A simple text answer.
- JSON Object: This format always returns a JSON object, with the structure provided as part of the prompt.
With this approach, we can deserialize the LLM’s response to an object that helps conditionally render the message content within the DevExpress Chat Component.
Structure
Here’s the structure I’m using:
public class MessageData
{
public string Message { get; set; }
public List Options { get; set; }
public string MessageTemplateName { get; set; }
}
public class OptionSet
{
public string Name { get; set; }
public string Description { get; set; }
public List Options { get; set; }
}
public class Option
{
public string Image { get; set; }
public string Url { get; set; }
public string Description { get; set; }
};
- MessageData: This structure will always be returned by our LLM.
- Option: A list of options for a message, which also serves as data for possible responses.
- OptionSet: A list of possible responses to feed into the prompt execution settings.
Prompt Execution Settings
One more step on the Semantic Kernel side is configuring the prompt execution settings:
var Settings = new OpenAIPromptExecutionSettings
{
MaxTokens = 500,
Temperature = 0.5,
ResponseFormat = "json_object"
};
Settings.ChatSystemPrompt = $"You need to answer using this JSON format with this structure {Structure} " +
$"Before giving an answer, check if it exists within this list of option sets {OptionSets}. " +
$"If your answer does not include options, the message template value should be 'Message'; otherwise, it should be 'Options'.";
In the prompt, we specify the structure {Structure}
we want as a response, provide a list of possible options for the message in the {OptionSets}
variable, and add a final line to guide the LLM on which template type to use.
Example Requests and Responses
For example, when executing the following request:
- Prompt: “Show me a list of Halloween costumes for cats.”
We’ll get this response from the LLM:
{
"Message": "Please select one of the Halloween costumes for cats",
"Options": [
{"Image": "./images/catblack.png", "Url": "https://cat.com/black", "Description": "Black cat costume"},
{"Image": "./images/catwhite.png", "Url": "https://cat.com/white", "Description": "White cat costume"},
{"Image": "./images/catorange.png", "Url": "https://cat.com/orange", "Description": "Orange cat costume"}
],
"MessageTemplateName": "Options"
}
With this JSON structure, we can conditionally render messages in the chat component as follows:
<DxAIChat CssClass="my-chat" MessageSent="MessageSent">
<MessageTemplate>
<div>
@{
if (@context.Typing)
{
<span>Loading...</span>
}
else
{
MessageData md = null;
try
{
md = JsonSerializer.Deserialize<MessageData>(context.Content);
}
catch
{
md = null;
}
if (md == null)
{
<div class="my-chat-content">
@context.Content
</div>
}
else
{
if (md.MessageTemplateName == "Options")
{
<div class="centered-carousel">
<Carousel class="carousel-container" Width="280" IsFade="true">
@foreach (var option in md.Options)
{
<CarouselItem>
<ChildContent>
<div>
<img src="@option.Image" alt="demo-image" />
<Button Color="Color.Primary" class="carousel-button">@option.Description</Button>
</div>
</ChildContent>
</CarouselItem>
}
</Carousel>
</div>
}
else if (md.MessageTemplateName == "Message")
{
<div class="my-chat-content">
@md.Message
</div>
}
}
}
}
</div>
</MessageTemplate>
</DxAIChat>
End Solution Example
Here’s an example of the final solution:
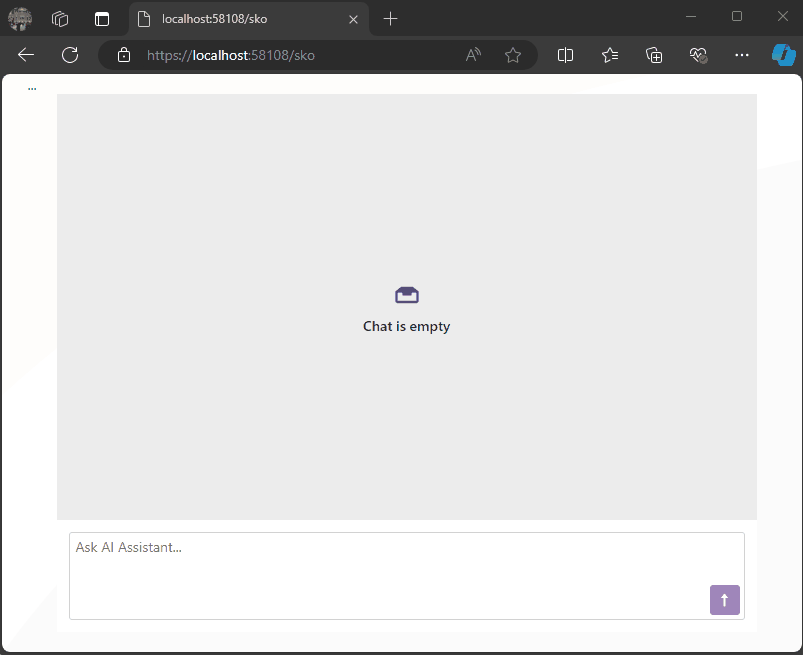
You can find the full source code here: https://github.com/egarim/devexpress-ai-chat-samples and a short video here https://youtu.be/dxMnOWbe3KA
by Joche Ojeda | Sep 4, 2024 | A.I, Semantic Kernel
In the world of AI and large language models (LLMs), understanding how to manage memory is crucial for creating applications that feel responsive and intelligent. Many developers are turning to Semantic Kernel, a lightweight and open-source development kit, to integrate these capabilities into their applications. For those already familiar with Semantic Kernel, let’s dive into how memory functions within this framework, especially when interacting with LLMs via chat completions.
Chat Completions: The Most Common Interaction with LLMs
When it comes to interacting with LLMs, one of the most intuitive and widely used methods is through chat completions. This allows developers to simulate a conversation between a user and an AI agent, facilitating various use cases like building chatbots, automating business processes, or even generating code.
In Semantic Kernel, chat completions are implemented through models from popular providers like OpenAI, Google, and others. These models enable developers to manage the flow of conversation seamlessly. While using chat completions, one key aspect to keep in mind is how the conversation history is stored and managed.
Temporary Memory: ChatHistory and Kernel String Arguments
Within the Semantic Kernel framework, the memory that a chat completion model uses is managed by the ChatHistory
object. This object stores the conversation history temporarily, meaning it captures the back-and-forth between the user and the model during an active session. Alternatively, you can use a string argument passed to the kernel, which contains context information for the conversation. However, like the ChatHistory
, this method is also not persistent.
Once the host class is disposed of, all stored context and memory from both the ChatHistory
object and the string argument are lost. This transient nature of memory means that these methods are useful only for short-term interactions and are destroyed after the session ends.
What’s Next? Exploring Long-Term Memory Options
In this article, we’ve discussed how Semantic Kernel manages short-term memory with ChatHistory
and kernel string arguments. However, for more complex applications that require retaining memory over longer periods—think customer support agents or business process automation—temporary memory might not be sufficient. In the next article, we’ll explore the options available for implementing long-term memory within Semantic Kernel, providing insights on how to make your AI applications even more powerful and context-aware.
Stay tuned for the deep dive into long-term memory solutions!
by Joche Ojeda | Jan 7, 2024 | A.I
Introduction
In the ever-evolving landscape of artificial intelligence, LangChain has emerged as a pivotal framework for harnessing the capabilities of large language models like GPT-3. This article delves into what LangChain is, its historical development, its applications, and concludes with its potential future impact.
What is LangChain?
LangChain is a software framework designed to facilitate the integration and application of advanced language models in various computational tasks. Developed by Shawn Presser, it stands as a testament to the growing need for accessible and versatile tools in the realm of AI and natural language processing (NLP). LangChain’s primary aim is to provide a modular and scalable environment where developers can easily implement and customize language models for a wide range of applications.
Historical Development
The Advent of Large Language Models
The genesis of LangChain is closely linked to the emergence of large language models. With the introduction of models like GPT-3 by OpenAI, the AI community witnessed a significant leap in the ability of machines to understand and generate human-like text.
Shawn Presser and LangChain
Recognizing the potential of these models, Shawn Presser embarked on developing a framework that would simplify their integration into practical applications. His vision led to the creation of LangChain, which he open-sourced to encourage community-driven development and innovation.
Applications
LangChain has found a wide array of applications, thanks to its versatile nature:
- Customer Service: By powering chatbots with nuanced and context-aware responses, LangChain enhances customer interaction and satisfaction.
- Content Creation: The framework assists in generating diverse forms of written content, from articles to scripts, offering tools for creativity and efficiency.
- Data Analysis: LangChain can analyze large volumes of text, providing insights and summaries, which are invaluable in research and business intelligence.
Conclusion
The story of LangChain is not just about a software framework; it’s about the democratization of AI technology. By making powerful language models more accessible and easier to integrate, LangChain is paving the way for a future where AI can be more effectively harnessed across various sectors. Its continued development and the growing community around it suggest a future rich with innovative applications, making LangChain a key player in the unfolding narrative of AI’s role in our world.
by Joche Ojeda | Jan 3, 2024 | A.I
Enhancing AI Language Models with Retrieval-Augmented Generation
Introduction
In the world of natural language processing and artificial intelligence, researchers and developers are constantly searching for ways to improve the capabilities of AI language models. One of the latest innovations in this field is Retrieval-Augmented Generation (RAG), a technique that combines the power of language generation with the ability to retrieve relevant information from a knowledge source. In this article, we will explore what RAG is, how it works, and its potential applications in various industries.
What is Retrieval-Augmented Generation?
Retrieval-Augmented Generation is a method that enhances AI language models by allowing them to access external knowledge sources to generate more accurate and contextually relevant responses. Instead of relying solely on the model’s internal knowledge, RAG enables the AI to retrieve relevant information from a database or a knowledge source, such as Wikipedia, and use that information to generate a response.
How does Retrieval-Augmented Generation work?
RAG consists of two main components: a neural retriever and a neural generator. The neural retriever is responsible for finding relevant information from the external knowledge source. It does this by searching for documents that are most similar to the input text or query. Once the relevant documents are retrieved, the neural generator processes the retrieved information and generates a response based on the context provided by the input text and the retrieved documents.
The neural retriever and the neural generator work together to create a more accurate and contextually relevant response. This combination allows the AI to produce higher-quality outputs and reduces the likelihood of generating incorrect or nonsensical information.
Potential Applications of Retrieval-Augmented Generation
Retrieval-Augmented Generation has a wide range of potential applications in various industries. Some of the most promising use cases include:
- Customer service: RAG can be used to improve the quality of customer service chatbots, allowing them to provide more accurate and relevant information to customers.
- Education: RAG can be used to create educational tools that provide students with accurate and up-to-date information on a wide range of topics.
- Healthcare: RAG can be used to develop AI systems that can assist doctors and healthcare professionals by providing accurate and relevant medical information.
- News and media: RAG can be used to create AI-powered news and media platforms that can provide users with accurate and contextually relevant information on current events and topics.
Conclusion
Retrieval-Augmented Generation is a powerful technique that has the potential to significantly enhance the capabilities of AI language models. By combining the power of language generation with the ability to retrieve relevant information from external sources, RAG can provide more accurate and contextually relevant responses. As the technology continues to develop, we can expect to see a wide range of applications for RAG in various industries.