by Joche Ojeda | Jul 3, 2024 | A.I, Blockchain, El Salvador
El Salvador, my birthplace, has recently emerged as a focal point for technological innovation under the leadership of President Nayib Bukele. Born in Suchitoto during the civil war and now living as a digital nomad in Saint Petersburg, Russia, I have witnessed El Salvador’s transformation from a distance and feel compelled to share its story. This article is the first in a series exploring how blockchain technology, financial services, and artificial intelligence (AI) can help a small country like El Salvador grow.
Historical Context and Economic Challenges
El Salvador has faced significant economic challenges over the past few decades, including poverty, gang violence, and a heavy reliance on remittances from abroad. The economy has traditionally been rooted in agriculture, with coffee and sugar being key exports. However, President Bukele, who took office on June 1, 2019, has sought to address these challenges by diversifying the economy and embracing technology as a key driver of growth.
Bukele’s Vision for Economic Transformation
President Bukele’s administration has prioritized technological innovation as a catalyst for economic transformation. His vision is to modernize the country’s infrastructure and position El Salvador as a hub for technological innovation in Latin America. This vision includes the strategic shift from an agriculture-based economy to one focused on technology, financial services, and tourism. The goal is to create a more resilient and diverse economic base that can sustain long-term growth and development.
The Adoption of Bitcoin as Legal Tender
One of the most groundbreaking moves by Bukele’s administration was the introduction of the Bitcoin Law, passed by the Legislative Assembly on June 9, 2021. This law made Bitcoin legal tender alongside the US dollar, which had been the country’s official currency since 2001. The rationale behind this decision was multifaceted:
- Financial Inclusion: With a significant portion of the population lacking access to traditional banking services, Bitcoin offers an alternative means of financial inclusion.
- Reduction in Remittance Costs: Remittances make up a substantial part of El Salvador’s economy. Bitcoin’s adoption aims to reduce the high transaction fees associated with remittance services.
- Economic Innovation: By adopting Bitcoin, El Salvador aims to attract foreign investment and position itself as a leader in cryptocurrency and blockchain technology.
The implementation of Bitcoin involved launching the Chivo Wallet, a state-sponsored digital wallet designed to facilitate Bitcoin transactions. The government also incentivized adoption by offering $30 worth of Bitcoin to citizens who registered for the wallet.
Initial Reactions and Impact
The reaction to the Bitcoin Law was mixed. While some praised the move as innovative and forward-thinking, others raised concerns about the volatility of Bitcoin and its potential impact on the economy. Despite these concerns, the Bukele administration has remained committed to its Bitcoin strategy, continuing to invest in Bitcoin and integrate it into the national economy.
Digital Transformation Initiatives
I
n addition to Bitcoin adoption, El Salvador has partnered with global tech giants like Google to enhance its digital infrastructure. These partnerships aim to modernize government services, improve healthcare through telemedicine platforms, and revolutionize education by integrating AI-driven tools. For instance, Google’s collaboration with the Salvadoran government includes training government agencies on cloud technologies and developing platforms that allow interoperability between institutions.
Strategic Shift to Technology, Financial Services, and Tourism
President Bukele’s broader economic strategy involves shifting El Salvador’s economic focus from traditional agriculture to more dynamic and sustainable sectors like technology, financial services, and tourism. This shift aims to create high-value jobs, attract foreign investment, and build a more diversified economy.
- Technology: By investing in digital infrastructure and fostering a favorable environment for tech startups, El Salvador aims to become a regional tech hub.
- Financial Services: The adoption of Bitcoin and other fintech innovations is intended to transform the financial landscape, making it more inclusive and efficient.
- Tourism: Enhancing the country’s tourism sector, with initiatives to promote its natural beauty and cultural heritage, is another key pillar of Bukele’s economic strategy.
Conclusion and Future Prospects
El Salvador’s journey towards becoming a technological leader in Latin America is a testament to the transformative power of visionary leadership and innovative policies. Under President Bukele, the country has taken bold steps to embrace technology, from adopting Bitcoin to integrating AI into public services. This series of articles will delve deeper into these initiatives, exploring their impact, challenges, and the future prospects for El Salvador in the global technological landscape.
By understanding El Salvador’s technological revolution, we can gain insights into the potential for other nations to leverage technology for economic and social development. The next article in this series will focus on the detailed implementation of Bitcoin as legal tender, examining the steps taken by the Bukele administration and the outcomes observed so far.
This introductory article sets the stage for a comprehensive exploration of El Salvador’s technological transformation under President Bukele. The subsequent articles will provide in-depth analyses and propose potential AI legislation to ensure the country’s continued leadership in technology within Latin America.
by Joche Ojeda | May 22, 2024 | A.I
A New Era of Computing: AI-Powered Devices Over Form Factor Innovations
In a recent Microsoft event, the spotlight was on a transformative innovation that highlights the power of AI over the constant pursuit of new device form factors. The unveiling of the new Surface computer, equipped with a Neural Processing Unit (NPU), demonstrates that enhancing existing devices with AI capabilities is more impactful than creating entirely new device types.
The Microsoft Event: Revolutionizing with AI
Microsoft showcased the new Surface computer, integrating an NPU that enhances performance by enabling real-time processing of AI algorithms on the device. This approach allows for advanced capabilities like enhanced voice recognition, real-time language translation, and sophisticated image processing, without relying on cloud services.
Why AI Integration Trumps New Form Factors
For years, the tech industry has focused on new device types, from tablets to foldable screens, often addressing problems that didn’t exist. However, the true advancement lies in making existing devices smarter. AI integration offers:
- Enhanced Productivity: Automating repetitive tasks and providing intelligent suggestions, allowing users to focus on more complex and creative work.
- Personalized Experience: Devices learn and adapt to user preferences, offering a highly customized experience.
- Advanced Capabilities: NPUs enable local processing of complex AI models, reducing latency and dependency on the cloud.
- Seamless Integration: AI creates a cohesive and efficient workflow across various applications and services.
Comparing to Humane Pin and Rabbit AI Devices
While devices like the Humane Pin and Rabbit AI offer innovative new form factors, they often rely heavily on cloud connectivity for AI functions. In contrast, the Surface’s NPU allows for faster, more secure local processing. This means tasks are completed quicker and more securely, as data doesn’t need to be sent to the cloud.
Conclusion: Embracing AI-Driven Innovation
Microsoft’s AI-enhanced Surface computer signifies a shift towards intelligent augmentation rather than just physical redesign. By embedding AI within existing devices, we unlock new potentials for efficiency, personalization, and functionality, setting a new standard for future tech innovations. This approach not only makes interactions with technology smarter and more intuitive but also emphasizes the importance of on-device processing power for a faster and more secure user experience.
For more information and to pre-order the new Surface laptops, visit Microsoft’s official store.
by Joche Ojeda | May 19, 2024 | A.I
OpenAI’s ChatGPT and Microsoft’s Copilot are two powerful AI tools that have revolutionized the way we interact with technology. While both are designed to assist users in various tasks, they each have unique features that set them apart.
OpenAI’s ChatGPT
ChatGPT, developed by OpenAI, is a large language model chatbot capable of communicating with users in a human-like way¹⁷. It can answer questions, create recipes, write code, and offer advice¹⁷. It uses a powerful generative AI model and has access to several tools which it can use to complete tasks²⁶.
Key Features of ChatGPT
- Chat with Images: You can show ChatGPT images and start a chat.
- Image Generation: Create images simply by describing them in ChatGPT.
- Voice Chat: You can now use voice to engage in a back-and-forth conversation with ChatGPT.
- Web Browsing: Gives ChatGPT the ability to search the internet for additional information.
- Advanced Data Analysis: Interact with data documents (Excel, CSV, JSON).
Microsoft’s Copilot
Microsoft’s Copilot is an AI companion that works everywhere you do and intelligently adapts to your needs. It can chat with text, voice, and image capabilities, summarize documents and web pages, create images, and use plugins and Copilot GPTs
Key Features of Copilot
- Chat with Text, Voice, and Image Capabilities: Copilot includes chat with text, voice, and image capabilities/
- Summarization of Documents and Web Pages: It can summarize documents and web pages.
- Image Creation: Copilot can create images.
- Web Grounding: It can ground information from the web.
- Use of Plugins and Copilot GPTs: Copilot can use plugins and Copilot GPTs.
Comparison of Mobile App Features
Feature |
OpenAI’s ChatGPT |
Microsoft’s Copilot |
Chat with Text |
Yes |
Yes |
Voice Input |
Yes |
Yes |
Image Capabilities |
Yes |
Yes |
Summarization |
No |
Yes |
Image Creation |
Yes |
Yes |
Web Grounding |
No |
Yes |
What makes the difference, the action button for the iPhone
The action button on iPhones, available on the iPhone 15 Pro and later models, is a customizable button for quick tasks. By default, it opens the camera or activates the flashlight. However, users can customize it to perform various actions, including launching a specific app. When set to launch an app, pressing the action button will instantly open the chosen app, such as the ChatGPT voice interface. This integration is further enhanced by the new ChatGPT-4.0 capabilities, which offer more accurate responses, better understanding of context, and faster processing times. This makes voice interactions with ChatGPT smoother and more efficient, allowing users to quickly and effectively communicate with the AI.
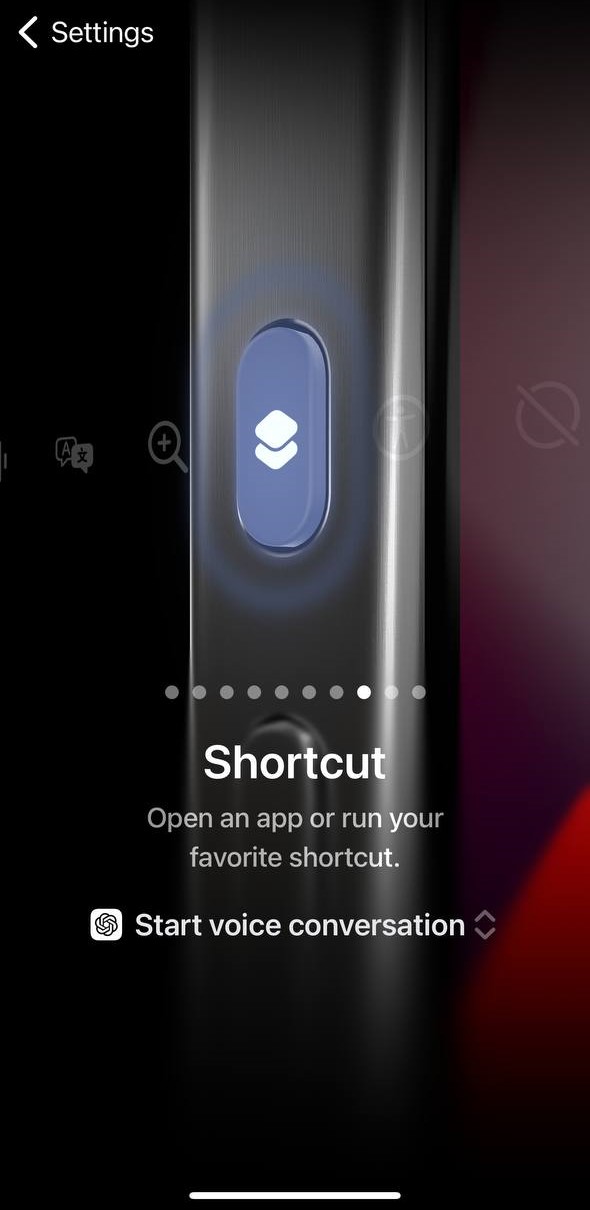 |
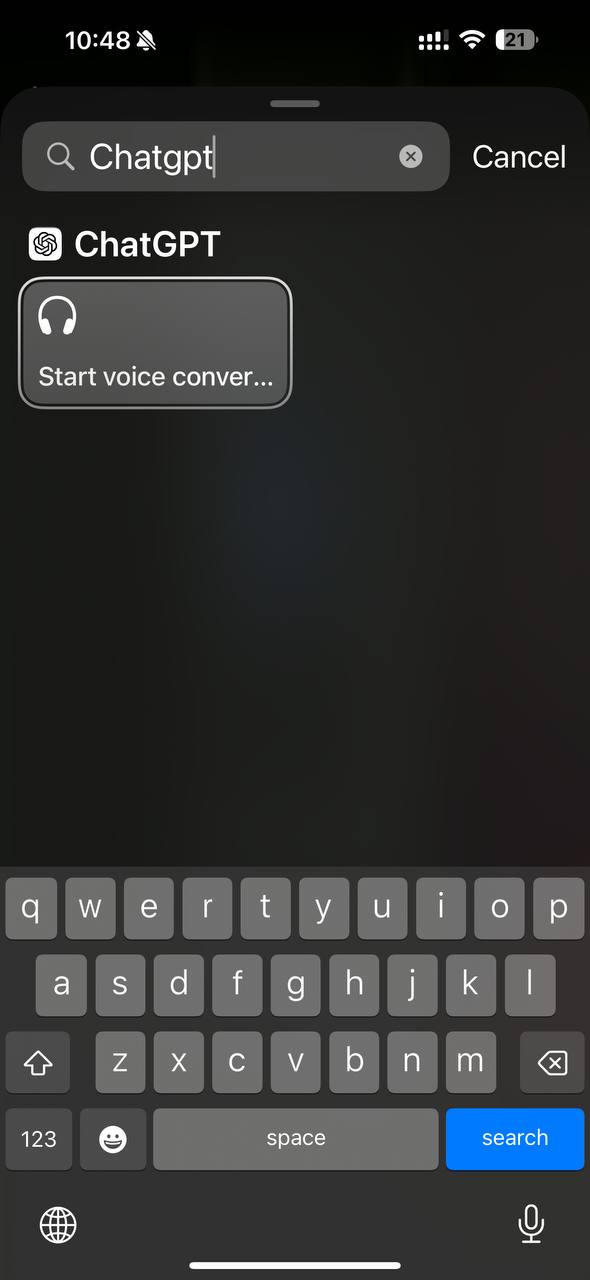 |
The ChatGPT voice interface is one of my favorite features, but there’s one thing missing for it to be perfect. Currently, you can’t send pictures or videos during a voice conversation. The workaround is to leave the voice interface, open the chat interface, find the voice conversation in the chat list, and upload the picture there. However, this brings another problem: you can’t return to the voice interface and continue the previous voice conversation.
Microsoft Copilot, if you are reading this, when will you add a voice interface? And when you finally do it, don’t forget to add the picture and video feature I want. That is all for my wishlist.
by Joche Ojeda | Jan 7, 2024 | A.I
Introduction
In the ever-evolving landscape of artificial intelligence, LangChain has emerged as a pivotal framework for harnessing the capabilities of large language models like GPT-3. This article delves into what LangChain is, its historical development, its applications, and concludes with its potential future impact.
What is LangChain?
LangChain is a software framework designed to facilitate the integration and application of advanced language models in various computational tasks. Developed by Shawn Presser, it stands as a testament to the growing need for accessible and versatile tools in the realm of AI and natural language processing (NLP). LangChain’s primary aim is to provide a modular and scalable environment where developers can easily implement and customize language models for a wide range of applications.
Historical Development
The Advent of Large Language Models
The genesis of LangChain is closely linked to the emergence of large language models. With the introduction of models like GPT-3 by OpenAI, the AI community witnessed a significant leap in the ability of machines to understand and generate human-like text.
Shawn Presser and LangChain
Recognizing the potential of these models, Shawn Presser embarked on developing a framework that would simplify their integration into practical applications. His vision led to the creation of LangChain, which he open-sourced to encourage community-driven development and innovation.
Applications
LangChain has found a wide array of applications, thanks to its versatile nature:
- Customer Service: By powering chatbots with nuanced and context-aware responses, LangChain enhances customer interaction and satisfaction.
- Content Creation: The framework assists in generating diverse forms of written content, from articles to scripts, offering tools for creativity and efficiency.
- Data Analysis: LangChain can analyze large volumes of text, providing insights and summaries, which are invaluable in research and business intelligence.
Conclusion
The story of LangChain is not just about a software framework; it’s about the democratization of AI technology. By making powerful language models more accessible and easier to integrate, LangChain is paving the way for a future where AI can be more effectively harnessed across various sectors. Its continued development and the growing community around it suggest a future rich with innovative applications, making LangChain a key player in the unfolding narrative of AI’s role in our world.