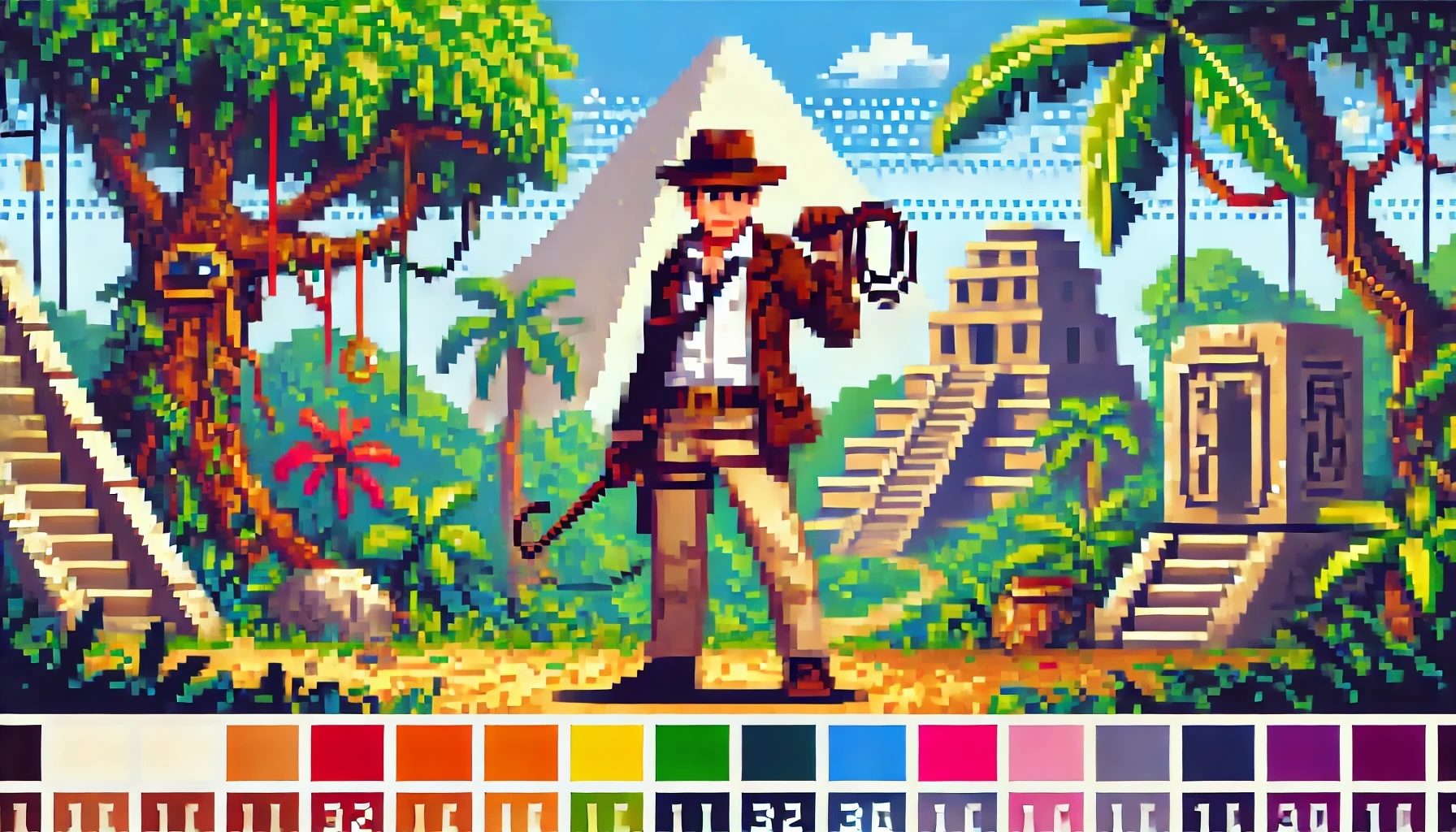
The mystery of lost values: Understanding ASCII vs. UTF-8 in Database Queries
Understanding ASCII vs. UTF-8 in Database Queries: A Practical Guide
When dealing with databases, understanding how different character encodings impact queries is crucial. Two common encoding standards are ASCII and UTF-8. This blog post delves into their differences, how they affect case-sensitive queries, and provides practical examples to illustrate these concepts.
ASCII vs. UTF-8: What’s the Difference?
ASCII (American Standard Code for Information Interchange)
- Description: A character encoding standard using 7 bits to represent each character, allowing for 128 unique symbols. These include control characters (like newline), digits, uppercase and lowercase English letters, and some special symbols.
- Range: 0 to 127.
UTF-8 (8-bit Unicode Transformation Format)
- Description: A variable-width character encoding capable of encoding all 1,112,064 valid character code points in Unicode using one to four 8-bit bytes. UTF-8 is backward compatible with ASCII.
- Range: Can represent characters in a much wider range, including all characters in all languages, as well as many symbols and special characters.
ASCII and UTF-8 Position Examples
Let’s compare the positions of some characters in both ASCII and UTF-8:
Character | ASCII Position | UTF-8 Position |
---|---|---|
A | 65 | 65 |
B | 66 | 66 |
… | … | … |
Y | 89 | 89 |
Z | 90 | 90 |
[ | 91 | 91 |
\ | 92 | 92 |
] | 93 | 93 |
^ | 94 | 94 |
_ | 95 | 95 |
` | 96 | 96 |
a | 97 | 97 |
b | 98 | 98 |
… | … | … |
y | 121 | 121 |
z | 122 | 122 |
Last ASCII (DEL) | 127 | 127 |
ÿ | Not present | 195 191 (2 bytes) |
Case Sensitivity in Database Queries
Case sensitivity can significantly impact database queries, as different encoding schemes represent characters differently.
ASCII Example
-- Case-sensitive query in ASCII-encoded database
SELECT * FROM users WHERE username = 'Alice';
-- This will not return rows with 'alice', 'ALICE', etc.
UTF-8 Example
-- Case-sensitive query in UTF-8 encoded database
SELECT * FROM users WHERE username = 'Ålice';
-- This will not return rows with 'ålice', 'ÅLICE', etc.
Practical Example with Positions
For ASCII, the characters included in the range >= 'A'
and <= 'z'
are:
A
has a position of 65.a
has a position of 97.
In a case-sensitive search, these positions are distinct, so A
is not equal to a
.
For UTF-8, the characters included in this range are the same since UTF-8 is backward compatible with ASCII for characters in this range.
Query Example
Let’s demonstrate a query example for usernames within the range >= 'A'
and <= 'z'
.
-- Query for usernames in the range 'A' to 'z'
SELECT * FROM users WHERE username >= 'A' AND username <= 'z';
Included Characters
Based on the ASCII positions, the range >= 'A'
and <= 'z'
includes:
- All uppercase letters:
A
toZ
(positions 65 to 90) - Special characters:
[
,\
,]
,^
,_
, and`
(positions 91 to 96) - All lowercase letters:
a
toz
(positions 97 to 122)
Practical Example with Positions
Given the following table:
-- Create a table
CREATE TABLE users (
id INT PRIMARY KEY,
username VARCHAR(255) CHARACTER SET utf8 COLLATE utf8_bin
);
-- Insert some users
INSERT INTO users (id, username) VALUES (1, 'Alice'); -- A = 65, l = 108, i = 105, c = 99, e = 101
INSERT INTO users (id, username) VALUES (2, 'alice'); -- a = 97, l = 108, i = 105, c = 99, e = 101
INSERT INTO users (id, username) VALUES (3, 'Ålice'); -- Å = 195 133, l = 108, i = 105, c = 99, e = 101
INSERT INTO users (id, username) VALUES (4, 'ålice'); -- å = 195 165, l = 108, i = 105, c = 99, e = 101
INSERT INTO users (id, username) VALUES (5, 'Z'); -- Z = 90
INSERT INTO users (id, username) VALUES (6, 'z'); -- z = 122
INSERT INTO users (id, username) VALUES (7, 'ÿ'); -- ÿ = 195 191
INSERT INTO users (id, username) VALUES (8, '_special');-- _ = 95, s = 115, p = 112, e = 101, c = 99, i = 105, a = 97, l = 108
INSERT INTO users (id, username) VALUES (9, 'example'); -- e = 101, x = 120, a = 97, m = 109, p = 112, l = 108, e = 101
Query Execution
-- Execute the query
SELECT * FROM users WHERE username >= 'A' AND username <= 'z';
Query Result
This query will include the following usernames based on the range:
Alice
(A = 65, l = 108, i = 105, c = 99, e = 101)Z
(Z = 90)example
(e = 101, x = 120, a = 97, m = 109, p = 112, l = 108, e = 101)_special
(_ = 95, s = 115, p = 112, e = 101, c = 99, i = 105, a = 97, l = 108)alice
(a = 97, l = 108, i = 105, c = 99, e = 101)z
(z = 122)
However, it will not include:
Ålice
(Å = 195 133, l = 108, i = 105, c = 99, e = 101, outside the specified range)ålice
(å = 195 165, l = 108, i = 105, c = 99, e = 101, outside the specified range)ÿ
(ÿ = 195 191, outside the specified range)
Conclusion
Understanding the differences between ASCII and UTF-8 character positions and ranges is crucial when performing case-sensitive queries in databases. For example, querying for usernames within the range >= 'A'
and <= 'z'
will include a specific set of characters based on their ASCII positions, impacting which rows are returned in your query results.
By grasping these concepts, you can ensure your database queries are accurate and efficient, especially when dealing with different encoding schemes.